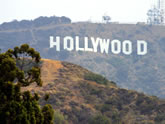
Movie
Donated on 7/6/1999
This data set contains a list of over 10000 films including many older, odd, and cult films. There is information on actors, casts, directors, producers, studios, etc.
Dataset Characteristics
Multivariate, Relational
Subject Area
Other
Associated Tasks
-
Feature Type
-
# Instances
10000
# Features
-
Dataset Information
Additional Information
The data is stored in relational form across several files. The central file (MAIN) is a list of movies, each with a unique identifier. These identifiers may change in successive versions. The actors (CAST) for those movies are listed with their roles in a distinct file. More information about individual actors (ACTORS) is in a third file. All directors in MAIN are listed in a fourth file (PEOPLE), with a number of important producers, writers, and cinematographers. A fifth file (REMAKES) links movies that were copied to a substantial extent from each other. The sixth file (STUDIOS) provides some information about studios shown in MAIN. The original motivation was for database class exercises, to replace the boring `manager of the toy-department' queries. Note that the CASTS, refering MAIN and ACTORS is logically identical to the inventory file refering to suppliers and assemblies in the the standard bill-of-materials problems. Personal interests caused the database to be made complete for all Hitchcock movies and TV episodes. Related films by type and actor were added gradually. Subsequent research on temporal databases caused date fields (years only) to be added. It allows testing, say, if the dates-of-work of an ACTOR match the dates of the MAIN films that the CAST relation shows. Object-oriented database features could be tested with fields having multiple and two-level values, as documented in DOC. The entries were gradually collected during course work starting about 1975 and are still being updated. Most of the entries were manual. The DOC file lists some of the reference works used. Corrections and additions continue to be appreciated. Detailed descriptions of the fields and their formats is provided in doc.html. Missing Values: Outside of key fields, missing values are common. Their encoding is described in DOC. Sometimes the data seems to be unavailable, sometimes it hasn't been entered. Some information, as `lived-with' is inherently incomplete. Censored Data: Minor actors are ignored. Dependencies: Every MAIN film must have a director in PEOPLE. About 50 pseudo director names ahve been listed in PEOPLE to allow interesting films to with (yet) unknown directors to be entered. Every CASTS entry must relate to a MAIN film entry. Every ACTOR should appear in some CASTS entry, but not vice versa. See DOC for more type information. Other Relevant Information: Films are listed, if known, with their original language title. An Alt(T: ) field provides English translations, where known. Data Format: The current files are in HTML, to allow easy parsing to other formats. An XML version is being considered. The approximate file sizes are: DOC ....... 50K MAIN ...... 1 145K 11 400 entries PEOPLE .... 355K 3 290 entries CASTS ..... 4 340K 46 000 entries ACTORS .... 811K 6 800 entries REMAKES ... 135K 1 278 entries STUDIOS ... 26K 200 entries
Has Missing Values?
Yes
Dataset Files
File | Size |
---|---|
data/casts.html | 4.2 MB |
data/main.html | 2 MB |
data/actors.html | 792.9 KB |
data/people.html | 347.6 KB |
data/adds.txt | 158.3 KB |
0 to 5 of 22
Papers Citing this Dataset
Sort by Year, desc
By Justin Chen, Edward Gan, Kexin Rong, Sahaana Suri, Peter Bailis. 2019
Published in ArXiv.
By Wenbo Gong, Sebastian Tschiatschek, Richard Turner, Sebastian Nowozin, Jos'e Hern'andez-Lobato, Cheng Zhang. 2019
Published in ArXiv.
By Armin Moharrer, Stratis Ioannidis. 2018
Published in IJCAI.
By Jiali Mei, Yohann Castro, Yannig Goude, Jean-Marc Azais, Georges H'ebrail. 2017
Published in IEEE Transactions on Knowledge and Data Engineering.
By Eric Nalisnick, Anima Anandkumar, Padhraic Smyth. 2015
Published in
0 to 5 of 6
Reviews
There are no reviews for this dataset yet.
pip install ucimlrepo
from ucimlrepo import fetch_ucirepo # fetch dataset movie = fetch_ucirepo(id=132) # data (as pandas dataframes) X = movie.data.features y = movie.data.targets # metadata print(movie.metadata) # variable information print(movie.variables)
Wiederhold, G. (1990). Movie [Dataset]. UCI Machine Learning Repository. https://doi.org/10.24432/C5SW2R.
Creators
Gio Wiederhold
DOI
License
This dataset is licensed under a Creative Commons Attribution 4.0 International (CC BY 4.0) license.
This allows for the sharing and adaptation of the datasets for any purpose, provided that the appropriate credit is given.