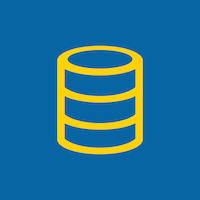
Micro Gas Turbine Electrical Energy Prediction
Donated on 4/23/2024
This dataset consists of measurements of electrical power corresponding to an input control signal over time, collected from a 3-kilowatt commercial micro gas turbine.
Dataset Characteristics
Sequential, Multivariate, Time-Series
Subject Area
Engineering
Associated Tasks
Regression
Feature Type
Real
# Instances
71225
# Features
1
Dataset Information
For what purpose was the dataset created?
Its original purpose was to learn the gas turbine's input-output temporal behavior with machine learning.
Are there recommended data splits?
In the original experiments we used experiments 1, 9, 20, 21, 23 and 24 for training and experiments 4 and 22 for testing. We used RMSE as the performance metric. See our referenced paper for experimental details.
Additional Information
The dataset comprises eight time series that depict the gas turbine’s behavior under diverse conditions. Each time series represents a separate experiment where the input control voltage was varied over time, and the resulting output electrical power of the micro gas turbine was measured. The time series vary in duration from 6,495 to 11,820 data points with a resolution of approximately 1 second, corresponding to approximately 1.8 to 3.3 hours. Each level of the input control signal corresponds to a stationary, i.e., constant, level of the output power. Notably, a noticeable delay occurs in the output signal during transitions in response to changes in the input control signal. There are rectangular and continuous time series: Four rectangular time series represent scenarios in which the input control signal changes instantaneously but the output power follows with a visible delay. Accurate modeling of both transitions and stationary phases is crucial for precise gas turbine modeling in these scenarios. The four remaining continuous time series represent scenarios in which the input control signal changes gradually and there are no visible delays in the output power. For these time series, learning transitions is less important for modeling the overall behavior.
Has Missing Values?
No
Introductory Paper
By Pawel Bielski, Aleksandr Eismont, Jakob Bach, Florian Leiser, Dustin Kottonau, and Klemens Böhm. 2024
Published in 15th ACM International Conference on Future Energy Systems (e-Energy '24), Singapore
Variables Table
Variable Name | Role | Type | Description | Units | Missing Values |
---|---|---|---|---|---|
time | ID | Continuous | Time | seconds | no |
input_voltage | Feature | Continuous | Input control voltage | volts | no |
el_power | Target | Continuous | Electrical output power | watts | no |
0 to 3 of 3
Dataset Files
File | Size |
---|---|
train.zip | 623.2 KB |
test.zip | 264.3 KB |
Reviews
There are no reviews for this dataset yet.
pip install ucimlrepo
from ucimlrepo import fetch_ucirepo # fetch dataset micro_gas_turbine_electrical_energy_prediction = fetch_ucirepo(id=994) # data (as pandas dataframes) X = micro_gas_turbine_electrical_energy_prediction.data.features y = micro_gas_turbine_electrical_energy_prediction.data.targets # metadata print(micro_gas_turbine_electrical_energy_prediction.metadata) # variable information print(micro_gas_turbine_electrical_energy_prediction.variables)
Bielski, P. & Kottonau, D. (2024). Micro Gas Turbine Electrical Energy Prediction [Dataset]. UCI Machine Learning Repository. https://doi.org/10.24432/C58S4T.
Keywords
Creators
Pawel Bielski
Karlsruhe Institute of Technology
Dustin Kottonau
Karlsruhe Institute of Technology
DOI
License
This dataset is licensed under a Creative Commons Attribution 4.0 International (CC BY 4.0) license.
This allows for the sharing and adaptation of the datasets for any purpose, provided that the appropriate credit is given.