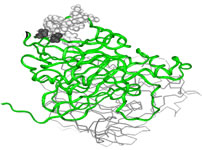
Dorothea
Donated on 2/28/2008
DOROTHEA is a drug discovery dataset. Chemical compounds represented by structural molecular features must be classified as active (binding to thrombin) or inactive. This is one of 5 datasets of the NIPS 2003 feature selection challenge.
Dataset Characteristics
Multivariate
Subject Area
Biology
Associated Tasks
Classification
Feature Type
Integer
# Instances
1950
# Features
100000
Dataset Information
Additional Information
Drugs are typically small organic molecules that achieve their desired activity by binding to a target site on a receptor. The first step in the discovery of a new drug is usually to identify and isolate the receptor to which it should bind, followed by testing many small molecules for their ability to bind to the target site. This leaves researchers with the task of determining what separates the active (binding) compounds from the inactive (non-binding) ones. Such a determination can then be used in the design of new compounds that not only bind, but also have all the other properties required for a drug (solubility, oral absorption, lack of side effects, appropriate duration of action, toxicity, etc.). The original data were modified for the purpose of the feature selection challenge. In particular, we added a number of distractor feature called 'probes' having no predictive power. The order of the features and patterns were randomized. DOROTHEA -- Positive ex. -- Negative ex. -- Total Training set -- 78 -- 722 -- 800 Validation set -- 34 -- 316 -- 350 Test set -- 78 -- 722 -- 800 All -- 190 -- 1760 -- 1950 We mapped Active compounds to the target value +1 (positive examples) and Inactive compounds to the target value –1 (negative examples). Number of variables/features/attributes: Real: 50000 Probes: 50000 Total: 100000 This dataset is one of five datasets used in the NIPS 2003 feature selection challenge. Our website http://www.nipsfsc.ecs.soton.ac.uk/ is still open for post-challenge submissions. Information about other related challenges are found at: http://clopinet.com/challenges. The CLOP package includes sample code to process these data: http://clopinet.com/CLOP. All details about the preparation of the data are found in our technical report: Design of experiments for the NIPS 2003 variable selection benchmark, Isabelle Guyon, July 2003, http://www.nipsfsc.ecs.soton.ac.uk/papers/NIPS2003-Datasets.pdf (also included in the dataset archive). Such information was made available only after the end of the challenge. The data are split into training, validation, and test set. Target values are provided only for the 2 first sets. Test set performance results are obtained by submitting prediction results to: http://www.nipsfsc.ecs.soton.ac.uk/. The data are in the following format: dataname.param: Parameters and statistics about the data dataname.feat: Identities of the features (withheld, to avoid biasing feature selection). dataname_train.data: Training set (a sparse binary matrix, patterns in lines, features in columns: the number of the non-zero features are provided). dataname_valid.data: Validation set. dataname_test.data: Test set. dataname_train.labels: Labels (truth values of the classes) for training examples. dataname_valid.labels: Validation set labels (withheld during the benchmark, but provided now). dataname_test.labels: Test set labels (withheld, so the data can still be use as a benchmark).
Has Missing Values?
No
Variable Information
We do not provide attribute information to avoid biasing feature selection.
Dataset Files
File | Size |
---|---|
DOROTHEA/dorothea_test.data | 4.1 MB |
DOROTHEA/dorothea_train.data | 4.1 MB |
DOROTHEA/dorothea_valid.data | 1.8 MB |
Dataset.pdf | 442.9 KB |
DOROTHEA/dorothea_train.labels | 2.3 KB |
0 to 5 of 7
Reviews
There are no reviews for this dataset yet.
pip install ucimlrepo
from ucimlrepo import fetch_ucirepo # fetch dataset dorothea = fetch_ucirepo(id=169) # data (as pandas dataframes) X = dorothea.data.features y = dorothea.data.targets # metadata print(dorothea.metadata) # variable information print(dorothea.variables)
Guyon, I., Gunn, S., Ben-Hur, A., & Dror, G. (2004). Dorothea [Dataset]. UCI Machine Learning Repository. https://doi.org/10.24432/C5NK6X.
Creators
Isabelle Guyon
Steve Gunn
Asa Ben-Hur
Gideon Dror
DOI
License
This dataset is licensed under a Creative Commons Attribution 4.0 International (CC BY 4.0) license.
This allows for the sharing and adaptation of the datasets for any purpose, provided that the appropriate credit is given.