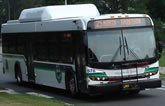
Statlog (Vehicle Silhouettes)
3D objects within a 2D image by application of an ensemble of shape feature extractors to the 2D silhouettes of the objects.
Dataset Characteristics
Multivariate
Subject Area
Other
Associated Tasks
Classification
Feature Type
Integer
# Instances
946
# Features
18
Dataset Information
Additional Information
The purpose is to classify a given silhouette as one of four types of vehicle, using a set of features extracted from the silhouette. The vehicle may be viewed from one of many different angles. HISTORY: This data was originally gathered at the TI in 1986-87 by JP Siebert. It was partially financed by Barr and Stroud Ltd. The original purpose was to find a method of distinguishing 3D objects within a 2D image by application of an ensemble of shape feature extractors to the 2D silhouettes of the objects. Measures of shape features extracted from example silhouettes of objects to be discriminated were used to generate a classification rule tree by means of computer induction. This object recognition strategy was successfully used to discriminate between silhouettes of model cars, vans and buses viewed from constrained elevation but all angles of rotation. The rule tree classification performance compared favourably to MDC (Minimum Distance Classifier) and k-NN (k-Nearest Neighbour) statistical classifiers in terms of both error rate and computational efficiency. An investigation of these rule trees generated by example indicated that the tree structure was heavily influenced by the orientation of the objects, and grouped similar object views into single decisions. DESCRIPTION: The features were extracted from the silhouettes by the HIPS (Hierarchical Image Processing System) extension BINATTS, which extracts a combination of scale independent features utilising both classical moments based measures such as scaled variance, skewness and kurtosis about the major/minor axes and heuristic measures such as hollows, circularity, rectangularity and compactness. Four "Corgie" model vehicles were used for the experiment: a double decker bus, Cheverolet van, Saab 9000 and an Opel Manta 400. This particular combination of vehicles was chosen with the expectation that the bus, van and either one of the cars would be readily distinguishable, but it would be more difficult to distinguish between the cars. The images were acquired by a camera looking downwards at the model vehicle from a fixed angle of elevation (34.2 degrees to the horizontal). The vehicles were placed on a diffuse backlit surface (lightbox). The vehicles were painted matte black to minimise highlights. The images were captured using a CRS4000 framestore connected to a vax 750. All images were captured with a spatial resolution of 128x128 pixels quantised to 64 greylevels. These images were thresholded to produce binary vehicle silhouettes, negated (to comply with the processing requirements of BINATTS) and thereafter subjected to shrink-expand-expand-shrink HIPS modules to remove "salt and pepper" image noise. The vehicles were rotated and their angle of orientation was measured using a radial graticule beneath the vehicle. 0 and 180 degrees corresponded to "head on" and "rear" views respectively while 90 and 270 corresponded to profiles in opposite directions. Two sets of 60 images, each set covering a full 360 degree rotation, were captured for each vehicle. The vehicle was rotated by a fixed angle between images. These datasets are known as e2 and e3 respectively. A further two sets of images, e4 and e5, were captured with the camera at elevations of 37.5 degs and 30.8 degs respectively. These sets also contain 60 images per vehicle apart from e4.van which contains only 46 owing to the difficulty of containing the van in the image at some orientations.
Has Missing Values?
No
Introductory Paper
Variables Table
Variable Name | Role | Type | Description | Units | Missing Values |
---|---|---|---|---|---|
COMPACTNESS | Feature | Integer | no | ||
CIRCULARITY | Feature | Integer | no | ||
DISTANCE CIRCULARITY | Feature | Integer | no | ||
RADIUS RATIO | Feature | Integer | no | ||
PR.AXIS ASPECT RATIO | Feature | Integer | no | ||
MAX.LENGTH ASPECT RATIO | Feature | Integer | no | ||
SCATTER RATIO | Feature | Integer | no | ||
ELONGATEDNESS | Feature | Integer | no | ||
PR.AXIS RECTANGULARITY | Feature | Integer | no | ||
MAX.LENGTH RECTANGULARITY | Feature | Integer | no |
0 to 10 of 19
Additional Variable Information
ATTRIBUTES COMPACTNESS (average perim)**2/area CIRCULARITY (average radius)**2/area DISTANCE CIRCULARITY area/(av.distance from border)**2 RADIUS RATIO (max.rad-min.rad)/av.radius PR.AXIS ASPECT RATIO (minor axis)/(major axis) MAX.LENGTH ASPECT RATIO (length perp. max length)/(max length) SCATTER RATIO (inertia about minor axis)/(inertia about major axis) ELONGATEDNESS area/(shrink width)**2 PR.AXIS RECTANGULARITY area/(pr.axis length*pr.axis width) MAX.LENGTH RECTANGULARITY area/(max.length*length perp. to this) SCALED VARIANCE (2nd order moment about minor axis)/area ALONG MAJOR AXIS SCALED VARIANCE (2nd order moment about major axis)/area ALONG MINOR AXIS SCALED RADIUS OF GYRATION (mavar+mivar)/area SKEWNESS ABOUT (3rd order moment about major axis)/sigma_min**3 MAJOR AXIS SKEWNESS ABOUT (3rd order moment about minor axis)/sigma_maj**3 MINOR AXIS KURTOSIS ABOUT (4th order moment about major axis)/sigma_min**4 MINOR AXIS KURTOSIS ABOUT (4th order moment about minor axis)/sigma_maj**4 MAJOR AXIS HOLLOWS RATIO (area of hollows)/(area of bounding polygon) Where sigma_maj**2 is the variance along the major axis and sigma_min**2 is the variance along the minor axis, and area of hollows= area of bounding poly-area of object The area of the bounding polygon is found as a side result of the computation to find the maximum length. Each individual length computation yields a pair of calipers to the object orientated at every 5 degrees. The object is propagated into an image containing the union of these calipers to obtain an image of the bounding polygon. NUMBER OF CLASSES 4 OPEL, SAAB, BUS, VAN
Baseline Model Performance
Reviews
There are no reviews for this dataset yet.
pip install ucimlrepo
from ucimlrepo import fetch_ucirepo # fetch dataset statlog_vehicle_silhouettes = fetch_ucirepo(id=149) # data (as pandas dataframes) X = statlog_vehicle_silhouettes.data.features y = statlog_vehicle_silhouettes.data.targets # metadata print(statlog_vehicle_silhouettes.metadata) # variable information print(statlog_vehicle_silhouettes.variables)
Mowforth,Pete and Shepherd,Barry. Statlog (Vehicle Silhouettes). UCI Machine Learning Repository. https://doi.org/10.24432/C5HG6N.
@misc{misc_statlog_(vehicle_silhouettes)_149, author = {Mowforth,Pete and Shepherd,Barry}, title = {{Statlog (Vehicle Silhouettes)}}, howpublished = {UCI Machine Learning Repository}, note = {{DOI}: https://doi.org/10.24432/C5HG6N} }
Creators
Pete Mowforth
Barry Shepherd
DOI
License
This dataset is licensed under a Creative Commons Attribution 4.0 International (CC BY 4.0) license.
This allows for the sharing and adaptation of the datasets for any purpose, provided that the appropriate credit is given.