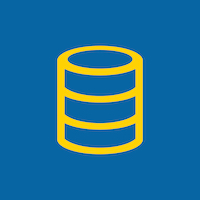
HEPMASS
Donated on 1/27/2016
The search for exotic particles requires sorting through a large number of collisions to find the events of interest. This data set challenges one to detect a new particle of unknown mass.
Dataset Characteristics
Multivariate
Subject Area
Physics and Chemistry
Associated Tasks
Classification
Feature Type
Real
# Instances
10500000
# Features
-
Dataset Information
Additional Information
Machine learning is used in high-energy physics experiments to search for the signatures of exotic particles. These signatures are learned from Monte Carlo simulations of the collisions that produce these particles and the resulting decay products. In each of the three data sets here, the goal is to separate particle-producing collisions from a background source. The mass of the new particle is unknown, so three separate data sets are provided. In each data set, 50% of the data is from a signal process, while 50% is from the background process. The data is separated into a training set of 7 million examples and a test set of 3.5 million for each. 1) In the '1000' dataset, the signal particle has mass=1000. (Note: this dataset does not include a mass feature since all signal examples have the same mass.) 2) In the 'not1000' dataset, the signal particle's mass is drawn uniformly from the set {500, 750, 1250, 1500}. The mass is included as an input feature; for the background examples, the mass is selected randomly from this same set. 3) In the 'all' dataset, the signal particle's mass is drawn uniformly from the set {500, 750, 1000, 1250, 1500}. The mass is included as an input feature; for the background examples, the mass is selected randomly from this same set.
Has Missing Values?
No
Variables Table
Variable Name | Role | Type | Description | Units | Missing Values |
---|---|---|---|---|---|
no | |||||
no | |||||
no | |||||
no | |||||
no | |||||
no | |||||
no | |||||
no | |||||
no | |||||
no |
0 to 10 of 28
Additional Variable Information
The first column is the class label (1 for signal, 0 for background), followed by the 27 normalized features (22 low-level features then 5 high-level features), and a 28th mass feature for datasets 2 and 3. See the original paper for more detailed information. There is a header line in each file.
Dataset Files
File | Size |
---|---|
all_train.csv.gz | 1.6 GB |
not1000_train.csv.gz | 1.6 GB |
1000_train.csv.gz | 1.6 GB |
all_test.csv.gz | 839.6 MB |
not1000_test.csv.gz | 838.9 MB |
0 to 5 of 6
Reviews
There are no reviews for this dataset yet.
pip install ucimlrepo
from ucimlrepo import fetch_ucirepo # fetch dataset hepmass = fetch_ucirepo(id=347) # data (as pandas dataframes) X = hepmass.data.features y = hepmass.data.targets # metadata print(hepmass.metadata) # variable information print(hepmass.variables)
Whiteson, D. (2016). HEPMASS [Dataset]. UCI Machine Learning Repository. https://doi.org/10.24432/C5PP5W.
Creators
Daniel Whiteson
DOI
License
This dataset is licensed under a Creative Commons Attribution 4.0 International (CC BY 4.0) license.
This allows for the sharing and adaptation of the datasets for any purpose, provided that the appropriate credit is given.