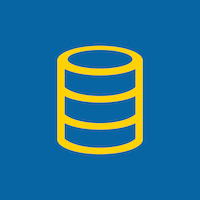
EEG Database
Donated on 10/12/1999
This data arises from a large study to examine EEG correlates of genetic predisposition to alcoholism. It contains measurements from 64 electrodes placed on the scalp sampled at 256 Hz
Dataset Characteristics
Multivariate, Time-Series
Subject Area
Health and Medicine
Associated Tasks
Classification
Feature Type
Categorical, Integer, Real
# Instances
122
# Features
-
Dataset Information
Additional Information
This data arises from a large study to examine EEG correlates of genetic predisposition to alcoholism. It contains measurements from 64 electrodes placed on subject's scalps which were sampled at 256 Hz (3.9-msec epoch) for 1 second. There were two groups of subjects: alcoholic and control. Each subject was exposed to either a single stimulus (S1) or to two stimuli (S1 and S2) which were pictures of objects chosen from the 1980 Snodgrass and Vanderwart picture set. When two stimuli were shown, they were presented in either a matched condition where S1 was identical to S2 or in a non-matched condition where S1 differed from S2. Shown here are example plots of a control (http://kdd.ics.uci.edu/databases/eeg/control.gif) and alcoholic (http://kdd.ics.uci.edu/databases/eeg/alcoholic.gif) subject. The plots indicate voltage, time, and channel and are averaged over 10 trials for the single stimulus condition. There were 122 subjects and each subject completed 120 trials where different stimuli were shown. The electrode positions were located at standard sites (Standard Electrode Position Nomenclature, American Electroencephalographic Association 1990). Zhang et al. (1995) describes in detail the data collection process. There are three versions of the EEG data set. 1. The Small Data Set The small data set (smni97_eeg_data.tar.gz) contains data for the 2 subjects, alcoholic a_co2a0000364 and control c_co2c0000337. For each of the 3 matching paradigms, c_1 (one presentation only), c_m (match to previous presentation) and c_n (no-match to previous presentation), 10 runs are shown. 2. The Large Data Set The large data set (SMNI_CMI_TRAIN.tar.gz and SMNI_CMI_TEST.tar.gz) contains data for 10 alcoholic and 10 control subjects, with 10 runs per subject per paradigm. The test data used the same 10 alcoholic and 10 control subjects as with the training data, but with 10 out-of-sample runs per subject per paradigm. 3. The Full Data Set This data set contains all 120 trials for 122 subjects. The entire set of data is about 700 MBytes. NOTE: There are 17 trials with empty files in co2c1000367. Some trials have "err" notices, e.g., search/grep for "err" and see "S2 match err" or "S2 nomatch err" etc.
Has Missing Values?
Yes
Variables Table
Variable Name | Role | Type | Description | Units | Missing Values |
---|---|---|---|---|---|
no | |||||
no | |||||
no | |||||
no |
0 to 4 of 4
Additional Variable Information
Each trial is stored in its own file and will appear in the following format. # co2a0000364.rd # 120 trials, 64 chans, 416 samples 368 post_stim samples # 3.906000 msecs uV # S1 obj , trial 0 # FP1 chan 0 0 FP1 0 -8.921 0 FP1 1 -8.433 0 FP1 2 -2.574 0 FP1 3 5.239 0 FP1 4 11.587 0 FP1 5 14.028 ... The first four lines are header information. Line 1 contains the subject identifier and indicates if the subject was an alcholic (a) or control (c) subject by the fourth letter. Line 4 identifies the matching conditions: a single object shown (S1 obj), object 2 shown in a matching condition (S2 match), and object 2 shown in a non matching condition (S2 nomatch). Line 5 identifies the start of the data from sensor FP1. The four columns of data are: the trial number, sensor position, sample number (0-255), and sensor value (in micro volts).
Dataset Files
File | Size |
---|---|
SMNI_CMI_TEST.tar.gz | 37 MB |
SMNI_CMI_TRAIN.tar.gz | 36.7 MB |
eeg_full/co2a0000419.tar.gz | 7.6 MB |
eeg_full/co3a0000450.tar.gz | 7.5 MB |
eeg_full/co2a0000369.tar.gz | 7.4 MB |
0 to 5 of 131
Papers Citing this Dataset
Sort by Year, desc
By Issam Laradji, Mark Schmidt, Vladimir Pavlovic, Minyoung Kim. 2019
Published in ArXiv.
By Kazuki Tachikawa, Yuji Kawai, Jihoon Park, Minoru Asada. 2018
Published in ArXiv.
By Michael Cohen, Jelena Diakonikolas, Lorenzo Orecchia. 2018
Published in ArXiv.
By Debarghya Ghoshdastidar, Ulrike Luxburg. 2018
Published in ArXiv.
0 to 5 of 20
Reviews
There are no reviews for this dataset yet.
pip install ucimlrepo
from ucimlrepo import fetch_ucirepo # fetch dataset eeg_database = fetch_ucirepo(id=121) # data (as pandas dataframes) X = eeg_database.data.features y = eeg_database.data.targets # metadata print(eeg_database.metadata) # variable information print(eeg_database.variables)
Begleiter, H. (1995). EEG Database [Dataset]. UCI Machine Learning Repository. https://doi.org/10.24432/C5TS3D.
Creators
Henri Begleiter
DOI
License
This dataset is licensed under a Creative Commons Attribution 4.0 International (CC BY 4.0) license.
This allows for the sharing and adaptation of the datasets for any purpose, provided that the appropriate credit is given.