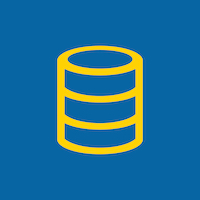
MicroMass
Donated on 8/11/2013
A dataset to explore machine learning approaches for the identification of microorganisms from mass-spectrometry data.
Dataset Characteristics
Multivariate
Subject Area
Biology
Associated Tasks
Classification
Feature Type
Real
# Instances
931
# Features
1300
Dataset Information
Additional Information
This MALDI-TOF dataset consists in: A) A reference panel of 20 Gram positive and negative bacterial species covering 9 genera among which several species are known to be hard to discriminate by mass spectrometry (MALDI-TOF). Each species was represented by 11 to 60 mass spectra obtained from 7 to 20 bacterial strains, constituting altogether a dataset of 571 spectra obtained from 213 strains. The spectra were obtained according to the standard culture-based workflow used in clinical routine in which the microorganism was first grown on an agar plate for 24 to 48 hours, before a portion of colony was picked, spotted on a MALDI slide and a mass spectrum was acquired. B) Based on this reference panel, a dedicated in vitro mock-up mixture dataset was constituted. For that purpose we considered 10 pairs of species of various taxonomic proximity: * 4 mixtures, labelled A, B, C and D, involved species that belong to the same genus, * 2 mixtures, labelled E and F, involved species that belong to distinct genera, but to the same Gram type, * 4 mixtures, labelled G, H, I and J, involved species that belong to distinct Gram types. Each mixture was represented by 2 pairs of strains, which were mixed according to the following 9 concentration ratios : 1:0, 10:1, 5:1, 2:1, 1:1, 1:2, 1:5, 1:10, 0:1. Two replicate spectra were acquired for each concentration ratio and each couple of strains, leading altogether to a dataset of 360 spectra, among which 80 are actually pure sample spectra.
Has Missing Values?
No
Dataset Files
File | Size |
---|---|
micromass_un_anonymized.zip | 770.6 KB |
pure_spectra_taxonomy.png | 54.4 KB |
Reviews
There are no reviews for this dataset yet.
pip install ucimlrepo
from ucimlrepo import fetch_ucirepo # fetch dataset micromass = fetch_ucirepo(id=253) # data (as pandas dataframes) X = micromass.data.features y = micromass.data.targets # metadata print(micromass.metadata) # variable information print(micromass.variables)
Mah, P. & Veyrieras, J. (2014). MicroMass [Dataset]. UCI Machine Learning Repository. https://doi.org/10.24432/C5T61S.
Creators
Pierre Mah
Jean-Baptiste Veyrieras
DOI
License
This dataset is licensed under a Creative Commons Attribution 4.0 International (CC BY 4.0) license.
This allows for the sharing and adaptation of the datasets for any purpose, provided that the appropriate credit is given.