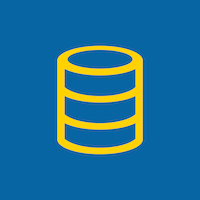
Online News Popularity
Donated on 5/30/2015
This dataset summarizes a heterogeneous set of features about articles published by Mashable in a period of two years. The goal is to predict the number of shares in social networks (popularity).
Dataset Characteristics
Multivariate
Subject Area
Business
Associated Tasks
Classification, Regression
Feature Type
Integer, Real
# Instances
39797
# Features
58
Dataset Information
Additional Information
* The articles were published by Mashable (www.mashable.com) and their content as the rights to reproduce it belongs to them. Hence, this dataset does not share the original content but some statistics associated with it. The original content be publicly accessed and retrieved using the provided urls. * Acquisition date: January 8, 2015 * The estimated relative performance values were estimated by the authors using a Random Forest classifier and a rolling windows as assessment method. See their article for more details on how the relative performance values were set.
Has Missing Values?
No
Introductory Paper
By Kelwin Fernandes, Pedro Vinagre, P. Cortez. 2015
Published in Portuguese Conference on Artificial Intelligence
Variables Table
Variable Name | Role | Type | Description | Units | Missing Values |
---|---|---|---|---|---|
url | ID | Categorical | no | ||
timedelta | Other | Continuous | no | ||
n_tokens_title | Feature | Continuous | no | ||
n_tokens_content | Feature | Continuous | no | ||
n_unique_tokens | Feature | Continuous | no | ||
n_non_stop_words | Feature | Continuous | no | ||
n_non_stop_unique_tokens | Feature | Continuous | no | ||
num_hrefs | Feature | Continuous | no | ||
num_self_hrefs | Feature | Continuous | no | ||
num_imgs | Feature | Continuous | no |
0 to 10 of 61
Additional Variable Information
Number of Attributes: 61 (58 predictive attributes, 2 non-predictive, 1 goal field) Attribute Information: 0. url: URL of the article (non-predictive) 1. timedelta: Days between the article publication and the dataset acquisition (non-predictive) 2. n_tokens_title: Number of words in the title 3. n_tokens_content: Number of words in the content 4. n_unique_tokens: Rate of unique words in the content 5. n_non_stop_words: Rate of non-stop words in the content 6. n_non_stop_unique_tokens: Rate of unique non-stop words in the content 7. num_hrefs: Number of links 8. num_self_hrefs: Number of links to other articles published by Mashable 9. num_imgs: Number of images 10. num_videos: Number of videos 11. average_token_length: Average length of the words in the content 12. num_keywords: Number of keywords in the metadata 13. data_channel_is_lifestyle: Is data channel 'Lifestyle'? 14. data_channel_is_entertainment: Is data channel 'Entertainment'? 15. data_channel_is_bus: Is data channel 'Business'? 16. data_channel_is_socmed: Is data channel 'Social Media'? 17. data_channel_is_tech: Is data channel 'Tech'? 18. data_channel_is_world: Is data channel 'World'? 19. kw_min_min: Worst keyword (min. shares) 20. kw_max_min: Worst keyword (max. shares) 21. kw_avg_min: Worst keyword (avg. shares) 22. kw_min_max: Best keyword (min. shares) 23. kw_max_max: Best keyword (max. shares) 24. kw_avg_max: Best keyword (avg. shares) 25. kw_min_avg: Avg. keyword (min. shares) 26. kw_max_avg: Avg. keyword (max. shares) 27. kw_avg_avg: Avg. keyword (avg. shares) 28. self_reference_min_shares: Min. shares of referenced articles in Mashable 29. self_reference_max_shares: Max. shares of referenced articles in Mashable 30. self_reference_avg_sharess: Avg. shares of referenced articles in Mashable 31. weekday_is_monday: Was the article published on a Monday? 32. weekday_is_tuesday: Was the article published on a Tuesday? 33. weekday_is_wednesday: Was the article published on a Wednesday? 34. weekday_is_thursday: Was the article published on a Thursday? 35. weekday_is_friday: Was the article published on a Friday? 36. weekday_is_saturday: Was the article published on a Saturday? 37. weekday_is_sunday: Was the article published on a Sunday? 38. is_weekend: Was the article published on the weekend? 39. LDA_00: Closeness to LDA topic 0 40. LDA_01: Closeness to LDA topic 1 41. LDA_02: Closeness to LDA topic 2 42. LDA_03: Closeness to LDA topic 3 43. LDA_04: Closeness to LDA topic 4 44. global_subjectivity: Text subjectivity 45. global_sentiment_polarity: Text sentiment polarity 46. global_rate_positive_words: Rate of positive words in the content 47. global_rate_negative_words: Rate of negative words in the content 48. rate_positive_words: Rate of positive words among non-neutral tokens 49. rate_negative_words: Rate of negative words among non-neutral tokens 50. avg_positive_polarity: Avg. polarity of positive words 51. min_positive_polarity: Min. polarity of positive words 52. max_positive_polarity: Max. polarity of positive words 53. avg_negative_polarity: Avg. polarity of negative words 54. min_negative_polarity: Min. polarity of negative words 55. max_negative_polarity: Max. polarity of negative words 56. title_subjectivity: Title subjectivity 57. title_sentiment_polarity: Title polarity 58. abs_title_subjectivity: Absolute subjectivity level 59. abs_title_sentiment_polarity: Absolute polarity level 60. shares: Number of shares (target)
Dataset Files
File | Size |
---|---|
OnlineNewsPopularity/OnlineNewsPopularity.csv | 23.2 MB |
OnlineNewsPopularity/OnlineNewsPopularity.names | 11.8 KB |
Papers Citing this Dataset
Sort by Year, desc
By Dariush Kari, Farhan Khan, Selami Ciftci, Suleyman Kozat. 2016
Published in
0 to 3 of 3
Reviews
There are no reviews for this dataset yet.
pip install ucimlrepo
from ucimlrepo import fetch_ucirepo # fetch dataset online_news_popularity = fetch_ucirepo(id=332) # data (as pandas dataframes) X = online_news_popularity.data.features y = online_news_popularity.data.targets # metadata print(online_news_popularity.metadata) # variable information print(online_news_popularity.variables)
Fernandes, K., Vinagre, P., Cortez, P., & Sernadela, P. (2015). Online News Popularity [Dataset]. UCI Machine Learning Repository. https://doi.org/10.24432/C5NS3V.
Creators
Kelwin Fernandes
Pedro Vinagre
Paulo Cortez
Pedro Sernadela
DOI
License
This dataset is licensed under a Creative Commons Attribution 4.0 International (CC BY 4.0) license.
This allows for the sharing and adaptation of the datasets for any purpose, provided that the appropriate credit is given.