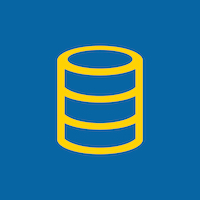
Stock Portfolio Performance
Donated on 4/21/2016
The data set of performances of weighted scoring stock portfolios are obtained with mixture design from the US stock market historical database.
Dataset Characteristics
Multivariate
Subject Area
Business
Associated Tasks
Regression
Feature Type
Real
# Instances
315
# Features
12
Dataset Information
Additional Information
There are three disadvantages of weighted scoring stock selection models. First, they cannot identify the relations between weights of stock-picking concepts and performances of portfolios. Second, they cannot systematically discover the optimal combination for weights of concepts to optimize the performances. Third, they are unable to meet various investors’ preferences. This study aims to more efficiently construct weighted scoring stock selection models to overcome these disadvantages. Since the weights of stock-picking concepts in a weighted scoring stock selection model can be regarded as components in a mixture, we used the simplex centroid mixture design to obtain the experimental sets of weights. These sets of weights are simulated with US stock market historical data to obtain their performances. Performance prediction models were built with the simulated performance data set and artificial neural networks. Furthermore, the optimization models to reflect investors’ preferences were built up, and the performance prediction models were employed as the kernel of the optimization models so that the optimal solutions can now be solved with optimization techniques. The empirical values of the performances of the optimal weighting combinations generated by the optimization models showed that they can meet various investors’ preferences and outperform those of S&P’s 500 not only during the training period but also during the testing period.
Has Missing Values?
No
Introductory Paper
By Yi-Cheng Liu, I. Yeh. 2017
Published in Neural computing & applications (Print)
Variables Table
Variable Name | Role | Type | Description | Units | Missing Values |
---|---|---|---|---|---|
period | ID | Categorical | no | ||
ID | ID | Integer | no | ||
Large B/P | Feature | Continuous | no | ||
Large ROE | Feature | Continuous | no | ||
Large S/P | Feature | Continuous | no | ||
Large Return Rate in the last quarter | Feature | Continuous | no | ||
Large Market Value | Feature | Continuous | no | ||
Small systematic Risk | Feature | Continuous | no | ||
Annual Return | Feature | Categorical | no | ||
Excess Return | Feature | Categorical | no |
0 to 10 of 20
Additional Variable Information
The inputs are the weights of the stock-picking concepts as follows X1=the weight of the Large B/P concept X2=the weight of the Large ROE concept X3=the weight of the Large S/P concept X4=the weight of the Large Return Rate in the last quarter concept X5=the weight of the Large Market Value concept X6=the weight of the Small systematic Risk concept The outputs are the investment performance indicators (normalized) as follows Y1=Annual Return Y2=Excess Return Y3=Systematic Risk Y4=Total Risk Y5=Abs. Win Rate Y6=Rel. Win Rate
Dataset Files
File | Size |
---|---|
stock portfolio performance data set.xlsx | 69.6 KB |
Reviews
There are no reviews for this dataset yet.
pip install ucimlrepo
from ucimlrepo import fetch_ucirepo # fetch dataset stock_portfolio_performance = fetch_ucirepo(id=390) # data (as pandas dataframes) X = stock_portfolio_performance.data.features y = stock_portfolio_performance.data.targets # metadata print(stock_portfolio_performance.metadata) # variable information print(stock_portfolio_performance.variables)
Yeh, I. (2015). Stock Portfolio Performance [Dataset]. UCI Machine Learning Repository. https://doi.org/10.24432/C5SC7G.
Creators
I-Cheng Yeh
DOI
License
This dataset is licensed under a Creative Commons Attribution 4.0 International (CC BY 4.0) license.
This allows for the sharing and adaptation of the datasets for any purpose, provided that the appropriate credit is given.