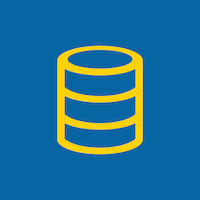
Data for Software Engineering Teamwork Assessment in Education Setting
Donated on 6/28/2017
Data include over 100 Team Activity Measures and outcomes (ML classes) obtained from activities of 74 student teams during the creation of final class project in SW Eng. classes at SFSU, Fulda, FAU
Dataset Characteristics
Sequential, Time-Series
Subject Area
Computer Science
Associated Tasks
Classification
Feature Type
Integer, Real
# Instances
74
# Features
-
Dataset Information
Additional Information
The data can be used to try to predict student learning in SE teamwork based on observation of their team activity **** README FILE from the submitted data ZIP **** # San Francisco State University # Software Engineering Team Assessment and Prediction (SETAP) Project # Machine Learning Training Data File Version 0.7 # ==================================================================== # # Copyright 2000-2017 by San Francisco State University, Dragutin # Petkovic, and Marc Sosnick-Perez. # # CONTACT # ------- # Professor Dragutin Petkovic: petkovic@sfsu.edu # # LICENSE # ------- # This data is released under the Creative Commons Attribution- # NonCommercial 4.0 International license. For more information, # please see # http://creativecommons.org/licenses/by-nc/4.0/legalcode. # # The research that has made this data possible has been funded in # part by NSF grant NSF-TUES1140172. # # YOUR FEEDBACK IS WELCOME # ------------------------ # We are interested in how this data is being used. If you use it in # a research project, we would like to know how you are using the # data. Please contact us at petkovic@sfsu.edu. # # # FILES INCLUDED IN DISTRIBUTION PACKAGE # ====================================== # This archive contains the data collected by the SETAP Project. # # # More data about the SETAP project, data collection, and description # and use of machine learning to analyze the data can be found in the # following paper: # # D. Petkovic, M. Sosnick-Perez, K. Okada, R. Todtenhoefer, S. Huang, # N. Miglani, A. Vigil: 'Using the Random Forest Classifier to Assess # and Predict Student Learning of Software Engineering Teamwork'. # Frontiers in Education FIE 2016, Erie, PA, 2016 # # # # See DATA DESCRIPTION below for more information about the data. The # README file (which you are reading) contains project information # such as data collection techniques, data organization and field # naming convention. In addition to the README file, the archive # contains a number of .csv files. Each of these CSV files contains # data aggregated by team from the project (see below), paired with # that team's outcome for either the process or product component of # the team's evaluation. The files are named using the following # convention: # # setap[Process|Product]T[1-11].csv # # For example, the file setapProcessT5.csv contains the data for all # teams for time interval 5, paired with the outcome data for the # Process component of the team's evaluation. # # Detailed information about the exact format of the .csv file may be # found in the csv files themselves. # # # DATA DESCRIPTION # ==================================================================== # The following is a detailed description of the data contained in the # accompanying files. # # INTRODUCTION # ------------ # # The data contained in these files were collected over a period of # several semesters from students engaged in software engineering # classes at San Francisco State University (class sections of CSC # 640, CSC 648 and CSC 848). All students consented to this data # being shared for research purposes provided no uniquely identifiable # information was contained in the distributed files. The information # was collected through various means, with emphasis being placed on # the collection of objective, quantifiable information. For more # information on the data collection procedures, please see the paper # referenced above. # # # PRIVACY # ------- # The data contained in this file does not contain any information # which may be individually traced to a particular student who # participated in the study. # # # BRIEF DESCRIPTION OF DATA SOURCES AND DERIVATIONS # ------------------------------------------------- # SAMs (Student Activity Measure) are collected for each student team # member during their participation in a software engineering class. # Student teams work together on a final class project, and comprise # 5-6 students. Teams that are made up of students from only one # school are labeled local teams. Teams made up of students from more # than one school are labeled global teams. SAMs are collected from: # weekly timecards, instructor observations, and software engineering # tool usage logs. SAMs are then aggregated by team and time interval # (see next section) into TAMs (Team Activity Measure). Outcomes are # determined at the end of the semester through evaluation of student # team work in two categories: software engineering process (how well # the team applied best software engineering practices), and software # engineering product (the quality of the finished product the team # produced). Thus for each team, two outcomes are determined, process # and product, respectively. Outcomes are classified into two class # grades, A or F. A represents teams that are at or above # expectations, F represents teams that are below expectations or need # attention. For more information, please see the paper referenced # above. # # The SE process and SE product outcomes represent ML training classes # and are to be considered separately, e.g. one should train ML for SE # process separately from training for SE product. # # TIME INTERVALS FOR WHICH DATA IS COLLECTED # ------------------------------------------ # Data collected continuously throughout the semester are aggregated # into different time intervals for the semester's project reflecting # different dynamics of teamwork during the class. Time intervals # represent time periods in which a milestone was developed by each # team. A milestone represents a major deliverable point in the class # for all student teams. The milestones are roughly divided into the # following topics: # # M1 - high level requirements and specs # M2 - more detailed requirements and specs # M3 - first prototype # M4 - beta release # M5 - final delivery # # Time intervals are combinations of the time in which milestones are # being produced. Time intervals are used in research only. # # In addition to time intervals corresponding to milestones, a number # of time intervals combining multiple T1-T5 time intervals have been # calculated. This was done to group student activities into design # vs. implementation phases which have different dynamics. # # These time intervals are defined as follows: # # Time Interval Corresponding Milestone Periods in Class # ----------------- -------------------------------------------- # 0 Milestone 0 # 1 Milestone 1 # 2 Milestone 2 # 3 Milestone 3 # 4 Milestone 4 # 5 Milestone 5 # 6 Milestone 1 - Milestone 2 inclusive # 7 Milestone 1 - Milestone 3 inclusive # 8 Milestone 1 - Milestone 4 inclusive # 9 Milestone 1 - Milestone 5 inclusive # 10 Milestone 4 - Milestone 5 inclusive # 11 Milestone 3 - Milestone 5 inclusive # # # # SETAP PROJECT OVERALL DATA STATISTICS # ================================================================== # The following is a set of statistics about the entire dataset which # may be useful in the configuration of machine learning methods. # # This data was collected only from students at SFSU. Global teams # represent only the data from the SFSU student portion of the team. # # GENERAL STATISTICS # ------------------ # Number of semesters: 7 # First semester: Fall 2012 # Last semester: Fall 2015 # Number of students: 383 # Class sections: 18 # # Number of TAM features: 115 # Number of class labels (outcomes): 2 # # Issues closed on time: 202 # Issues closed late: + 53 # ------- # Total issues: 255 # # TEAM COMPOSITION STATISTCS # -------------------------- # Local Teams: 59 # Global Teams: + 15 # ------ # Total: 74 Teams # # OUTCOME (CLASSIFICATION) STATISTICS # ----------------------------------- # Total Outcomes: 74 # # Proces Product # ------------------ ------------------ # outcome: A F A F # 49 25 42 32 # # TAM FEATURE NAMING CONVENTION # ----------------------------- # A systematic approach to aggregating and naming TAM features was # developed. By using this systematic approach, TAM feature names are # produced that are human understandable and intuitive and related to # aggregation method. # # # There are a number of base TAM which are then aggregated into # aggregated TAM. # # BASE TAM # -------- # # General TAM # ----------- # The following TAMs are collected for each team: Year, semester, # timeInterval, teamNumber, semesterId, teamMemberCount, # femaleTeamMembersPercent, teamLeadGender, teamDistribution # # Calculated TAM # -------------- # For each team, TAM were calculated from SAMs for every time interval # Ti. The core TAM variables where for each we compute as applicable: # count, average, standard deviation over weeks, over students etc. # # TAMs collected by Weekly Time Cards (WTS) TAM # --------------------------------------------- # teamMemberResponseCount, meetingHours, inPersonMeetingHours. # nonCodingDeliverablesHours, codingDeliverablesHours, helpHours, # globalLeadAdminHours, LeadAdminHoursResponseCount, # GlobalLeadAdminHoursResponseCount # # TAMs collected by Tool Logs (TL) TAM # ------------------------------------- # commitCount, uniqueCommitMessageCount, uniqueCommitMessagePercent, # CommitMessageLength # # Collected by Instructor Observations (IO) TAMs # ------------------------------------------------ # issueCount, onTimeIssueCount, lateIssueCount # # # AGGREGATED TAM # -------------- # # Several aggregation method and derived variable names for TAMs # reflect how the core TAM variables were aggregated in final TAM # measures for each time interval Ti: # # Let VAR be the core TAM variable above. The naming conventions and # aggregation operators to obtain TAMs for each time interval Ti were # as follows: # # <VAR>Total - total sum of VAR in the time interval Ti # <VAR>Average - average of VAR in the time interval # <VAR>StandardDeviation - SD of variable in time interval # <VAR>Count - count of events measured by VAR (e.g. missed # checkpoints) in time interval # Average<VAR>ByWeek - total sum/count of VAR in the time interval # divided by weeks in time interval # StandradDeviation<VAR>ByWeek - the standard devation of the weekly # total of VAR taken over the time interval # Average<VAR>ByStudent - total count/sum of VAR in time interval, # divided by number of students in the team # StandardDeviation <VAR>ByStudent - standard deviation of VAR in the # time interval, over students in the team # # # NULL VALUES # ----------- # NULL values are used in the training data to indicate that no SAMs # were recorded in that particular time period, week, or for that # student. # # Frequently TAM features involving teamLeadHours or globalTeamLead # hours will result in a NULL for a particular training sample. For # local team leads, that usually means that the local team lead did # not complete any timecard surveys for the aggregation in quesiton. # While for global team lead TAM features this may also be the case, # the more usual cause of NULLS in global team lead TAM features comes # from the fact that most teams are not global, and therefore this # statistic was not gathered for these teams. # # It is left to the individual researcher to decide how to accomodate # NULL values, and the data is included in this file. Though these # may not be useful for machine learning directly, valuable # information can be obatined with some processing. # # TAM FEATURES # ------------ # The following is a list of tam features available in the data files. # The TAM feature names are listed in the order in which the data # appear in each training sample, i.e. the first feature corresponds # to the first column, the second feature corresponds to the second # column, etc. # # The first sample line in the data section of the data file is not a # true sample, but consists of TAM feature names, which allows for # easy import into spreadsheets and for human readability. # # The final two TAM features (columns) are the outcome data for # process and product, and are the last two columns in each sample # row. The training sample data follow the header comment section. # # # TAM FEATURE LIST # ---------------- # year # semester # timeInterval # teamNumber # semesterId # teamMemberCount # femaleTeamMembersPercent # teamLeadGender # teamDistribution # teamMemberResponseCount # meetingHoursTotal # meetingHoursAverage # meetingHoursStandardDeviation # inPersonMeetingHoursTotal # inPersonMeetingHoursAverage # inPersonMeetingHoursStandardDeviation # nonCodingDeliverablesHoursTotal # nonCodingDeliverablesHoursAverage # nonCodingDeliverablesHoursStandardDeviation # codingDeliverablesHoursTotal # codingDeliverablesHoursAverage # codingDeliverablesHoursStandardDeviation # helpHoursTotal # helpHoursAverage # helpHoursStandardDeviation # leadAdminHoursResponseCount # leadAdminHoursTotal # leadAdminHoursAverage # leadAdminHoursStandardDeviation # globalLeadAdminHoursResponseCount # globalLeadAdminHoursTotal # globalLeadAdminHoursAverage # globalLeadAdminHoursStandardDeviation # averageResponsesByWeek # standardDeviationResponsesByWeek # averageMeetingHoursTotalByWeek # standardDeviationMeetingHoursTotalByWeek # averageMeetingHoursAverageByWeek # standardDeviationMeetingHoursAverageByWeek # averageInPersonMeetingHoursTotalByWeek # standardDeviationInPersonMeetingHoursTotalByWeek # averageInPersonMeetingHoursAverageByWeek # standardDeviationInPersonMeetingHoursAverageByWeek # averageNonCodingDeliverablesHoursTotalByWeek # standardDeviationNonCodingDeliverablesHoursTotalByWeek # averageNonCodingDeliverablesHoursAverageByWeek # standardDeviationNonCodingDeliverablesHoursAverageByWeek # averageCodingDeliverablesHoursTotalByWeek # standardDeviationCodingDeliverablesHoursTotalByWeek # averageCodingDeliverablesHoursAverageByWeek # standardDeviationCodingDeliverablesHoursAverageByWeek # averageHelpHoursTotalByWeek # standardDeviationHelpHoursTotalByWeek # averageHelpHoursAverageByWeek # standardDeviationHelpHoursAverageByWeek # averageLeadAdminHoursResponseCountByWeek # standardDeviationLeadAdminHoursResponseCountByWeek # averageLeadAdminHoursTotalByWeek # standardDeviationLeadAdminHoursTotalByWeek # averageGlobalLeadAdminHoursResponseCountByWeek # standardDeviationGlobalLeadAdminHoursResponseCountByWeek # averageGlobalLeadAdminHoursTotalByWeek # standardDeviationGlobalLeadAdminHoursTotalByWeek # averageGlobalLeadAdminHoursAverageByWeek # standardDeviationGlobalLeadAdminHoursAverageByWeek # averageResponsesByStudent # standardDeviationResponsesByStudent # averageMeetingHoursTotalByStudent # standardDeviationMeetingHoursTotalByStudent # averageMeetingHoursAverageByStudent # standardDeviationMeetingHoursAverageByStudent # averageInPersonMeetingHoursTotalByStudent # standardDeviationInPersonMeetingHoursTotalByStudent # averageInPersonMeetingHoursAverageByStudent # standardDeviationInPersonMeetingHoursAverageByStudent # averageNonCodingDeliverablesHoursTotalByStudent # standardDeviationNonCodingDeliverablesHoursTotalByStudent # averageNonCodingDeliverablesHoursAverageByStudent # standardDeviationNonCodingDeliverablesHoursAverageByStudent # averageCodingDeliverablesHoursTotalByStudent # standardDeviationCodingDeliverablesHoursTotalByStudent # averageCodingDeliverablesHoursAverageByStudent # standardDeviationCodingDeliverablesHoursAverageByStudent # averageHelpHoursTotalByStudent # standardDeviationHelpHoursTotalByStudent # averageHelpHoursAverageByStudent # standardDeviationHelpHoursAverageByStudent # commitCount # uniqueCommitMessageCount # uniqueCommitMessagePercent # commitMessageLengthTotal # commitMessageLengthAverage # commitMessageLengthStandardDeviation # averageCommitCountByWeek # standardDeviationCommitCountByWeek # averageUniqueCommitMessageCountByWeek # standardDeviationUniqueCommitMessageCountByWeek # averageUniqueCommitMessagePercentByWeek # standardDeviationUniqueCommitMessagePercentByWeek # averageCommitMessageLengthTotalByWeek # standardDeviationCommitMessageLengthTotalByWeek # averageCommitCountByStudent # standardDeviationCommitCountByStudent # averageUniqueCommitMessageCountByStudent # standardDeviationUniqueCommitMessageCountByStudent # averageUniqueCommitMessagePercentByStudent # standardDeviationUniqueCommitMessagePercentByStudent # averageCommitMessageLengthTotalByStudent # standardDeviationCommitMessageLengthTotalByStudent # averageCommitMessageLengthAverageByStudent # standardDeviationCommitMessageLengthAverageByStudent # averageCommitMessageLengthStandardDeviationByStudent # issueCount # onTimeIssueCount # lateIssueCount # processLetterGrade # productLetterGrade
Has Missing Values?
Yes
Variables Table
Variable Name | Role | Type | Description | Units | Missing Values |
---|---|---|---|---|---|
no | |||||
no | |||||
no | |||||
no | |||||
no | |||||
no | |||||
no | |||||
no | |||||
no | |||||
no |
0 to 10 of 102
Additional Variable Information
See above
Reviews
There are no reviews for this dataset yet.
pip install ucimlrepo
from ucimlrepo import fetch_ucirepo # fetch dataset data_for_software_engineering_teamwork_assessment_in_education_setting = fetch_ucirepo(id=393) # data (as pandas dataframes) X = data_for_software_engineering_teamwork_assessment_in_education_setting.data.features y = data_for_software_engineering_teamwork_assessment_in_education_setting.data.targets # metadata print(data_for_software_engineering_teamwork_assessment_in_education_setting.metadata) # variable information print(data_for_software_engineering_teamwork_assessment_in_education_setting.variables)
Petkovic,D., Todtenhoefer,Rainer, and Huang,Shihong. (2017). Data for Software Engineering Teamwork Assessment in Education Setting. UCI Machine Learning Repository. https://doi.org/10.24432/C5BK5R.
@misc{misc_data_for_software_engineering_teamwork_assessment_in_education_setting_393, author = {Petkovic,D., Todtenhoefer,Rainer, and Huang,Shihong}, title = {{Data for Software Engineering Teamwork Assessment in Education Setting}}, year = {2017}, howpublished = {UCI Machine Learning Repository}, note = {{DOI}: https://doi.org/10.24432/C5BK5R} }
Creators
D. Petkovic
Rainer Todtenhoefer
Shihong Huang
DOI
License
This dataset is licensed under a Creative Commons Attribution 4.0 International (CC BY 4.0) license.
This allows for the sharing and adaptation of the datasets for any purpose, provided that the appropriate credit is given.