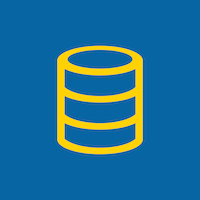
Electrical Grid Stability Simulated Data
Donated on 11/15/2018
The local stability analysis of the 4-node star system (electricity producer is in the center) implementing Decentral Smart Grid Control concept.
Dataset Characteristics
Multivariate
Subject Area
Physics and Chemistry
Associated Tasks
Classification, Regression
Feature Type
Real
# Instances
10000
# Features
12
Dataset Information
Additional Information
The analysis is performed for different sets of input values using the methodology similar to that described in [Schäfer, Benjamin, et al. 'Taming instabilities in power grid networks by decentralized control.' The European Physical Journal Special Topics 225.3 (2016): 569-582.]. Several input values are kept the same: averaging time: 2 s; coupling strength: 8 s^-2; damping: 0.1 s^-1
Has Missing Values?
No
Variables Table
Variable Name | Role | Type | Description | Units | Missing Values |
---|---|---|---|---|---|
tau1 | Feature | Continuous | no | ||
tau2 | Feature | Continuous | no | ||
tau3 | Feature | Continuous | no | ||
tau4 | Feature | Continuous | no | ||
p1 | Feature | Continuous | no | ||
p2 | Feature | Continuous | no | ||
p3 | Feature | Continuous | no | ||
p4 | Feature | Continuous | no | ||
g1 | Feature | Continuous | no | ||
g2 | Feature | Continuous | no |
0 to 10 of 14
Additional Variable Information
11 predictive attributes, 1 non-predictive(p1), 2 goal fields: 1. tau[x]: reaction time of participant (real from the range [0.5,10]s). Tau1 - the value for electricity producer. 2. p[x]: nominal power consumed(negative)/produced(positive)(real). For consumers from the range [-0.5,-2]s^-2; p1 = abs(p2 + p3 + p4) 3. g[x]: coefficient (gamma) proportional to price elasticity (real from the range [0.05,1]s^-1). g1 - the value for electricity producer. 4. stab: the maximal real part of the characteristic equation root (if positive - the system is linearly unstable)(real) 5. stabf: the stability label of the system (categorical: stable/unstable)
Dataset Files
File | Size |
---|---|
Data_for_UCI_named.csv | 2.3 MB |
Reviews
There are no reviews for this dataset yet.
pip install ucimlrepo
from ucimlrepo import fetch_ucirepo # fetch dataset electrical_grid_stability_simulated_data = fetch_ucirepo(id=471) # data (as pandas dataframes) X = electrical_grid_stability_simulated_data.data.features y = electrical_grid_stability_simulated_data.data.targets # metadata print(electrical_grid_stability_simulated_data.metadata) # variable information print(electrical_grid_stability_simulated_data.variables)
Arzamasov, V. (2018). Electrical Grid Stability Simulated Data [Dataset]. UCI Machine Learning Repository. https://doi.org/10.24432/C5PG66.
Creators
Vadim Arzamasov
DOI
License
This dataset is licensed under a Creative Commons Attribution 4.0 International (CC BY 4.0) license.
This allows for the sharing and adaptation of the datasets for any purpose, provided that the appropriate credit is given.