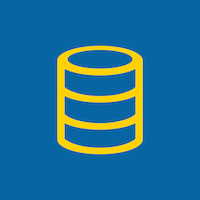
Image Recognition Task Execution Times in Mobile Edge Computing
Donated on 8/14/2023
Recorded task execution times for four Edge Servers submitted by edge node; node sends images to servers for image recognition tasks. The servers perform the tasks and return the results to nodes.
Dataset Characteristics
Sequential, Time-Series
Subject Area
Computer Science
Associated Tasks
Regression
Feature Type
Real
# Instances
4000
# Features
2
Dataset Information
Additional Information
This dataset contains the turnaround execution times (in seconds) for offloaded image recognition tasks when executed in different edge servers. The edge servers are MacBook Pro Processor: 1.4 GHz Quad-Core Intel Core i5 RAM: 8 GB 2133 MHz LPDDR3, MacBook Pro Processor: 2.5 GHz Dual-Core Intel Core i5 RAM: 8 GB 1600 MHz DDR3, Ubuntu VM Using VirtualBox RAM: 2 GB and Raspberry Pi 4B Processor: a quad-core 64-bit ARM Cortex-A72 CPU RAM: 4Gb. The client (mobile edge node) was simulated as a process in one of these devices. The client sends an image to be recognized by one of the servers above, i.e. server. The turnaround execution time is the time duration once the connection is established (when the edge node starts sending the image) until it receives the recognition result from the edge server. The execution time is recorded for each edge server.
Has Missing Values?
No
Introductory Paper
By Ibrahim A. Alghamdi, C. Anagnostopoulos, D. Pezaros. 2019
Published in Wireless Days
Variable Information
[1] Time: day, date, hours, minutes, second, year. [2] Turnaround Task Execution time: in seconds.
Dataset Files
File | Size |
---|---|
TATDescriptionDataset.zip | 79 KB |
Reviews
There are no reviews for this dataset yet.
pip install ucimlrepo
from ucimlrepo import fetch_ucirepo # fetch dataset image_recognition_task_execution_times_in_mobile_edge_computing = fetch_ucirepo(id=859) # data (as pandas dataframes) X = image_recognition_task_execution_times_in_mobile_edge_computing.data.features y = image_recognition_task_execution_times_in_mobile_edge_computing.data.targets # metadata print(image_recognition_task_execution_times_in_mobile_edge_computing.metadata) # variable information print(image_recognition_task_execution_times_in_mobile_edge_computing.variables)
Alghamdi, I., Anagnostopoulos, C., & Pezaros, D. (2019). Image Recognition Task Execution Times in Mobile Edge Computing [Dataset]. UCI Machine Learning Repository. https://doi.org/10.24432/C5N617.
Keywords
Creators
Ibrahim Alghamdi
i.alghamdi.1@research.gla.ac.uk
Christos Anagnostopoulos
christos.anagnostopoulos@glasgow.ac.uk
Dimitrios Pezaros
dimitrios.pezaros@glasgow.ac.uk
DOI
License
This dataset is licensed under a Creative Commons Attribution 4.0 International (CC BY 4.0) license.
This allows for the sharing and adaptation of the datasets for any purpose, provided that the appropriate credit is given.