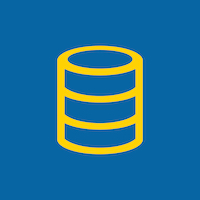
REJAFADA
Donated on 8/14/2023
REJAFADA (Retrieval of Jar Files Applied to Dynamic Analysis) aims to be used, as benchmark, to check the quality of the detection of Jar malware.
Dataset Characteristics
Multivariate
Subject Area
Computer Science
Associated Tasks
Classification
Feature Type
Integer
# Instances
1996
# Features
6825
Dataset Information
Additional Information
The REJAFADA (Retrieval of Jar Files Applied to Dynamic Analysis) is a dataset which allows the classification of files with Jar extension between benign and malwares. The REJAFADA is composed of 998 malware Jar files and 998 other benign Jar files. The REJAFADA dataset, consequently, is suitable for learning endowed with AI (Artificial Intelligence), considering that the Jar files presented the same amount in the different classes (malware and benign). The goal is that tendentious classifiers, in relation to a certain class, do not have their success taxes favored. In relation to virtual plagues, REJAFADA extracted malicious Jar files from VirusShare which is a repository of malware samples to provide security researchers, incident responders, forensic analysts, and the morbidly curious access to samples of live malicious code. With respect to benign Jar files, the catalog was given from application repositories such as Java2s.com, and findar.com. All of the benign files have been audited by VirusTotal. Then, the benign Jar files, contained in REJAFADA, had their benevolence attested by the main commercial antiviruses of the world. The obtained results corresponding to the analyses of the benign and malware Jar files, resulting from the VirusTotal audit, are available for consultation at the virtual address of REJAFADA ¹. The features of Jar files originate through the dynamic analysis of suspicious files. Therefore, in our methodology, the malware is executed in order to infect, intentionally, the Java Virtual Machine installed in Windows 7 audited, in real time (dynamic), by the Cuckoo Sandbox. 1. REJAFADA (A Retrieval of Jar Files Applied to Dynamic Analysis). Available in: https://github.com/rewema/rejafada. Accessed on June 2018.
Has Missing Values?
No
Introductory Paper
By Ricardo P Pinheiro, Sidney M. L. Lima, Sérgio M. M. Fernandes, E. D. Q. Albuquerque, S. Medeiros, Danilo Souza, T. Monteiro, Petrônio Lopes, Rafael Lima, Jemerson Oliveira, Sthéfano Silva. 2019
Published in International Conference on Computer Supported Cooperative Work in Design
Variable Information
1) Application name 2) Class (M = malware, B = benign) 3) Input Attribute (3-6826). Next, the groups of features are detailed - Features related to virtual machines. - Features related to malware. - Features related to Backdoors. - Features related to the banking threats (Trojan horses). - Features related to Bitcoin. - Features related to bots (machines that perform automatic network tasks, malicious or not, without the knowledge of their owners). - Features related to browsers. - Features related to Firewall. - Features related to cloud computing. - Features related to DDoS (Dynamic Danial of Service) attacks. - Features that seek to disable features of Windows 7 OS and other utilities. - Features associated with network traffic hint windows 7 OS in PCAP format. - Features related to DNS servers (Domain Name System, servers responsible for the translation of URL addresses in IP). - Features related to native Windows 7 OS programs. - Features related to Windows 7 Boot OS. - Features related to Windows 7 OS (Regedit). - Features related to the use of sandboxes. The digital forensics examines whether the file tried tries to detect whether sandboxes: Cuckoo, Joe, Anubis, Sunbelt, ThreatTrack / GFI / CW or Fortinet are being used, through the presence of their own files. - Features related to antivirus. Checks if the file being investigated tries to check for registry keys, in regedit, for Chinese antivirus. - Features related to Ransomware (type of malware that by means of encryption, leaves the victim's files unusable, then request a redemption in exchange for the normal use later of the user's files, a redemption usually paid in a non-traceable way, such as bitcoins). - Features related to exploit-related features which constitute malware attempting to exploit known or unackaged vulnerabilities, faults or defects in the system or one or more of its components in order to cause unforeseen instabilities and behavior on both your hardware and in your software. - Features related to Infostealers, malicious programs that collect confidential information from the affected computer.
Dataset Files
File | Size |
---|---|
REJAFADA.zip | 365.8 KB |
Reviews
There are no reviews for this dataset yet.
pip install ucimlrepo
from ucimlrepo import fetch_ucirepo # fetch dataset rejafada = fetch_ucirepo(id=860) # data (as pandas dataframes) X = rejafada.data.features y = rejafada.data.targets # metadata print(rejafada.metadata) # variable information print(rejafada.variables)
Pinheiro, R., M. L. de Lima, S., Murilo, S., Albuquerque, E., Souza, D., Monteiro, T., Lopes, P., Lima, R., Oliveira, J., & Silva, S. (2019). REJAFADA [Dataset]. UCI Machine Learning Repository. https://doi.org/10.24432/C5HG8D.
Keywords
Creators
Ricardo Pinheiro
Sidney M. L. de Lima
sidney.lima@ufpe.br
Federal University of Pernambuco
Sérgio Murilo
Edison Albuquerque
Danilo Souza
Thyago Monteiro
Petrônio Lopes
Rafael Lima
Jemerson Oliveira
Sthéfano Silva
DOI
License
This dataset is licensed under a Creative Commons Attribution 4.0 International (CC BY 4.0) license.
This allows for the sharing and adaptation of the datasets for any purpose, provided that the appropriate credit is given.