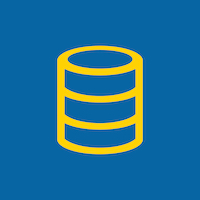
Anonymous Microsoft Web Data
Donated on 10/31/1998
Log of anonymous users of www.microsoft.com; predict areas of the web site a user visited based on data on other areas the user visited.
Dataset Characteristics
-
Subject Area
Computer Science
Associated Tasks
Recommender-Systems
Feature Type
Categorical
# Instances
37711
# Features
294
Dataset Information
Additional Information
We created the data by sampling and processing the www.microsoft.com logs. The data records the use of www.microsoft.com by 38000 anonymous, randomly-selected users. For each user, the data lists all the areas of the web site (Vroots) that user visited in a one week timeframe. Users are identified only by a sequential number, for example, User #14988, User #14989, etc. The file contains no personally identifiable information. The 294 Vroots are identified by their title (e.g. "NetShow for PowerPoint") and URL (e.g. "/stream"). The data comes from one week in February, 1998.
Has Missing Values?
No
Variable Information
Each attribute is an area ("vroot") of the www.microsoft.com web site. The datasets record which Vroots each user visited in a one-week timeframe in Feburary 1998.
Dataset Files
File | Size |
---|---|
anonymous-msweb.data | 1.4 MB |
anonymous-msweb.test | 223.2 KB |
anonymous-msweb.info | 3.6 KB |
Papers Citing this Dataset
Sort by Year, desc
By Yueqin Hu, Raymond Treinen. 2019
Published in The British journal of mathematical and statistical psychology.
By Mehmet Hacibeyoglu, Mohammed Ibrahim. 2018
Published in Scientific Programming.
By Xiangbing Zhou, Fang Miao, Hongjiang Ma. 2018
Published in Information.
By Wenzhang Zhuge, Chenping Hou, Yuanyuan Jiao, Jia Yue, Hong Tao, Dongyun Yi. 2017
Published in PloS one.
By Tianyu Wang, Marco Morucci, M. Awan, Yameng Liu, Sudeepa Roy, Cynthia Rudin, Alexander Volfovsky. 2017
Published in ArXiv.
0 to 5 of 8
Reviews
There are no reviews for this dataset yet.
pip install ucimlrepo
from ucimlrepo import fetch_ucirepo # fetch dataset anonymous_microsoft_web_data = fetch_ucirepo(id=4) # data (as pandas dataframes) X = anonymous_microsoft_web_data.data.features y = anonymous_microsoft_web_data.data.targets # metadata print(anonymous_microsoft_web_data.metadata) # variable information print(anonymous_microsoft_web_data.variables)
Breese, J., Heckerman, D., & Kadie, C. (1998). Anonymous Microsoft Web Data [Dataset]. UCI Machine Learning Repository. https://doi.org/10.24432/C5VS3Q.
Keywords
Creators
Jack Breese
David Heckerman
Carl Kadie
DOI
License
This dataset is licensed under a Creative Commons Attribution 4.0 International (CC BY 4.0) license.
This allows for the sharing and adaptation of the datasets for any purpose, provided that the appropriate credit is given.