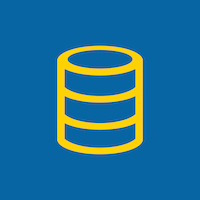
Caesarian Section Classification Dataset
Donated on 11/1/2018
This dataset contains information about caesarian section results of 80 pregnant women with the most important characteristics of delivery problems in the medical field.
Dataset Characteristics
Tabular
Subject Area
Health and Medicine
Associated Tasks
Classification
Feature Type
Integer
# Instances
80
# Features
-
Dataset Information
Has Missing Values?
No
Variables Table
Variable Name | Role | Type | Description | Units | Missing Values |
---|---|---|---|---|---|
no | |||||
no | |||||
no | |||||
no | |||||
no |
0 to 5 of 5
Additional Variable Information
We choose age, delivery number, delivery time, blood pressure and heart status. We classify delivery time to Premature, Timely and Latecomer. As like the delivery time we consider blood pressure in three statuses of Low, Normal and High moods. Heart Problem is classified as apt and inept. @attribute 'Age' { 22,26,28,27,32,36,33,23,20,29,25,37,24,18,30,40,31,19,21,35,17,38 } @attribute 'Delivery number' { 1,2,3,4 } @attribute 'Delivery time' { 0,1,2 } -> {0 = timely , 1 = premature , 2 = latecomer} @attribute 'Blood of Pressure' { 2,1,0 } -> {0 = low , 1 = normal , 2 = high } @attribute 'Heart Problem' { 1,0 } -> {0 = apt, 1 = inept } @attribute Caesarian { 0,1 } -> {0 = No, 1 = Yes }
Dataset Files
File | Size |
---|---|
caesarian.csv.arff | 1.4 KB |
Reviews
There are no reviews for this dataset yet.
pip install ucimlrepo
from ucimlrepo import fetch_ucirepo # fetch dataset caesarian_section_classification_dataset = fetch_ucirepo(id=472) # data (as pandas dataframes) X = caesarian_section_classification_dataset.data.features y = caesarian_section_classification_dataset.data.targets # metadata print(caesarian_section_classification_dataset.metadata) # variable information print(caesarian_section_classification_dataset.variables)
Amin, M. & Ali, A. (2018). Caesarian Section Classification Dataset [Dataset]. UCI Machine Learning Repository. https://doi.org/10.24432/C5N59X.
Creators
Muhammad Amin
Amir Ali
DOI
License
This dataset is licensed under a Creative Commons Attribution 4.0 International (CC BY 4.0) license.
This allows for the sharing and adaptation of the datasets for any purpose, provided that the appropriate credit is given.