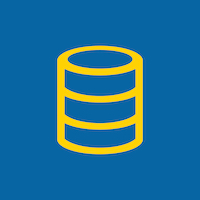
Crowdsourced Mapping
Donated on 5/24/2016
Crowdsourced data from OpenStreetMap is used to automate the classification of satellite images into different land cover classes (impervious, farm, forest, grass, orchard, water).
Dataset Characteristics
Multivariate
Subject Area
Climate and Environment
Associated Tasks
Classification
Feature Type
-
# Instances
10546
# Features
29
Dataset Information
Additional Information
This dataset was derived from geospatial data from two sources: 1) Landsat time-series satellite imagery from the years 2014-2015, and 2) crowdsourced georeferenced polygons with land cover labels obtained from OpenStreetMap. The crowdsourced polygons cover only a small part of the image area, and are used used to extract training data from the image for classifying the rest of the image. The main challenge with the dataset is that both the imagery and the crowdsourced data contain noise (due to cloud cover in the images and innaccurate labeling/digitizing of polygons). Files in zip folder -The 'training.csv' file contains the training data for classification. Do not use this file to evaluate classification accuracy because it contains noise (many class labeling errors). -The 'testing.csv' file contains testing data to evaluate the classification accuracy. This file does not contain any class labeling errors.
Has Missing Values?
No
Variable Information
class: The land cover class (impervious, farm, forest, grass, orchard, water) [note: this is the target variable to classify]. max_ndvi: the maximum NDVI (normalized difference vegetation index) value derived from the time-series of satellite images. 20150720_N - 20140101_N : NDVI values extracted from satellite images acquired between January 2014 and July 2015, in reverse chronological order (dates given in the format yyyymmdd).
Dataset Files
File | Size |
---|---|
training.csv | 2.3 MB |
testing.csv | 67.9 KB |
Reviews
There are no reviews for this dataset yet.
pip install ucimlrepo
from ucimlrepo import fetch_ucirepo # fetch dataset crowdsourced_mapping = fetch_ucirepo(id=400) # data (as pandas dataframes) X = crowdsourced_mapping.data.features y = crowdsourced_mapping.data.targets # metadata print(crowdsourced_mapping.metadata) # variable information print(crowdsourced_mapping.variables)
Johnson, B. (2016). Crowdsourced Mapping [Dataset]. UCI Machine Learning Repository. https://doi.org/10.24432/C56315.
Creators
Brian Johnson
DOI
License
This dataset is licensed under a Creative Commons Attribution 4.0 International (CC BY 4.0) license.
This allows for the sharing and adaptation of the datasets for any purpose, provided that the appropriate credit is given.