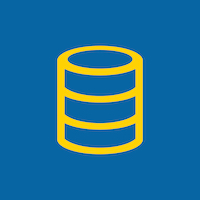
Forest type mapping
Donated on 5/24/2015
Multi-temporal remote sensing data of a forested area in Japan. The goal is to map different forest types using spectral data.
Dataset Characteristics
Multivariate
Subject Area
Biology
Associated Tasks
Classification
Feature Type
-
# Instances
326
# Features
27
Dataset Information
Additional Information
This data set contains training and testing data from a remote sensing study which mapped different forest types based on their spectral characteristics at visible-to-near infrared wavelengths, using ASTER satellite imagery. The output (forest type map) can be used to identify and/or quantify the ecosystem services (e.g. carbon storage, erosion protection) provided by the forest.
Has Missing Values?
No
Variable Information
Class: 's' ('Sugi' forest), 'h' ('Hinoki' forest), 'd' ('Mixed deciduous' forest), 'o' ('Other' non-forest land) b1 - b9: ASTER image bands containing spectral information in the green, red, and near infrared wavelengths for three dates (Sept. 26, 2010; March 19, 2011; May 08, 2011. pred_minus_obs_S_b1 - pred_minus_obs_S_b9: Predicted spectral values (based on spatial interpolation) minus actual spectral values for the 's' class (b1-b9). pred_minus_obs_H_b1 - pred_minus_obs_H_b9: Predicted spectral values (based on spatial interpolation) minus actual spectral values for the 'h' class (b1-b9).
Dataset Files
File | Size |
---|---|
testing.csv | 45.4 KB |
training.csv | 27.8 KB |
Reviews
There are no reviews for this dataset yet.
pip install ucimlrepo
from ucimlrepo import fetch_ucirepo # fetch dataset forest_type_mapping = fetch_ucirepo(id=333) # data (as pandas dataframes) X = forest_type_mapping.data.features y = forest_type_mapping.data.targets # metadata print(forest_type_mapping.metadata) # variable information print(forest_type_mapping.variables)
Johnson, B. (2012). Forest type mapping [Dataset]. UCI Machine Learning Repository. https://doi.org/10.24432/C5QP56.
Creators
Brian Johnson
DOI
License
This dataset is licensed under a Creative Commons Attribution 4.0 International (CC BY 4.0) license.
This allows for the sharing and adaptation of the datasets for any purpose, provided that the appropriate credit is given.