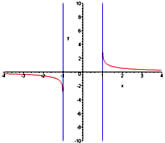
Function Finding
Donated on 8/31/1990
Cases collected mostly from investigations in physical science; intention is to evaluate function-finding algorithms
Dataset Characteristics
-
Subject Area
Physics and Chemistry
Associated Tasks
Function-Learning
Feature Type
Real
# Instances
352
# Features
-
Dataset Information
Additional Information
[Please note the use of Latex format here for algebraic expressions. See Leslie Lamport, Latex: A Document Preparation System, Addison-Wesley, 1986 for details.] This database contains 352 bivariate numeric data sets collected from diverse sources and resulting, with a few exceptions, from investigations in physical science. For each data set, the collection includes: 1. Source: Bibliographic information for the source of the data. 2. Description: Identification of the variables $x$ and $y$. Except in a few clearly identified instances, the abbreviated format $y$ vs. $x$ is employed. An entry of the form Description: Force vs. separation. indicates that $x$ is a separation and $y$ is a force. In some cases--when the information was readily available--the description also includes the units in which the data was originally reported. 3. Reference relation: The functional relationship proposed by the reporting scientist in the original source. 4. Comments (optional): Additional information pertaining to the case. In recording reference relations, the database often omits details of parameter values. If a scientist proposes $y=23.1x-.0014$, the reference relation may be given as just $y=k_{1}x+k_{2}$. Also, since algebraic transformations have been employed freely, the same relation might be given as $y/x=k_{2}/x+k_{1}$. In general, data collected here is given in full as it appeared in the original source. Fractions have been converted to decimals, numbers have been freely translated to and from scientific notation and zeros have sometimes been added to decimal numbers to facilitate tabulation. Any additional deviations from verbatim transcription are noted in the Comments entry of the associated case. Note in particular that, in a few clearly identified cases, apparent typographical errors have been corrected and that, in others, data points identified by the reporting scientist as *not* conforming to the proposed relationship have been omitted.
Has Missing Values?
No
Dataset Files
File | Size |
---|---|
function-finding.data | 211.5 KB |
function-finding.names | 8.4 KB |
Index | 141 Bytes |
Reviews
There are no reviews for this dataset yet.
pip install ucimlrepo
from ucimlrepo import fetch_ucirepo # fetch dataset function_finding = fetch_ucirepo(id=41) # data (as pandas dataframes) X = function_finding.data.features y = function_finding.data.targets # metadata print(function_finding.metadata) # variable information print(function_finding.variables)
Schaffer, C. (1990). Function Finding [Dataset]. UCI Machine Learning Repository. https://doi.org/10.24432/C57W35.
Creators
Cullen Schaffer
DOI
License
This dataset is licensed under a Creative Commons Attribution 4.0 International (CC BY 4.0) license.
This allows for the sharing and adaptation of the datasets for any purpose, provided that the appropriate credit is given.