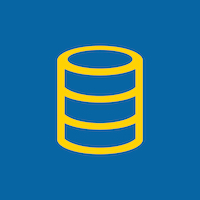
Gas Sensor Array Drift at Different Concentrations
Donated on 10/22/2013
This archive contains 13910 measurements from 16 chemical sensors exposed to 6 different gases at various concentration levels.
Dataset Characteristics
Multivariate, Time-Series
Subject Area
Computer Science
Associated Tasks
Classification, Regression, Clustering, Causa
Feature Type
Real
# Instances
13910
# Features
128
Dataset Information
Additional Information
This data set contains 13,910 measurements from 16 chemical sensors exposed to 6 gases at different concentration levels. This dataset is an extension of the Gas Sensor Array Drift Dataset (http://archive.ics.uci.edu/ml/datasets/Gas+Sensor+Array+Drift+Dataset), providing now the information about the concentration level at which the sensors were exposed for each measurement. The primary purpose of making this dataset freely accessible on-line is to provide an extensive dataset to the sensor and artificial intelligence research communities to develop and test strategies to solve a wide variety of tasks, including sensor drift, classification, regression, among others. The dataset can be used exclusively for research purposes. Commercial purposes are fully excluded. Citation of both Vergara et al. 'Chemical gas sensor drift compensation using classifier ensembles' and Rodriguez-Lujan et al. “On the calibration of sensor arrays for pattern recognition using the minimal number of experiments†is required (see below). The dataset was gathered during the period of January 2008 to February 2011 (36 months) in a gas delivery platform facility situated at the ChemoSignals Laboratory in the BioCircuits Institute, University of California San Diego. The measurement system platform provides versatility for obtaining the desired concentrations of the chemical substances of interest with high accuracy and in a highly reproducible manner, minimizing thereby the common mistakes caused by human intervention and making it possible to exclusively concentrate on the chemical sensors. See reference 1 for more details on the experimental setup. The resulting dataset comprises recordings from six distinct pure gaseous substances, namely Ammonia, Acetaldehyde, Acetone, Ethylene, Ethanol, and Toluene, dosed at a wide variety of concentration levels in the intervals (50,1000), (5,500), (12,1000), (10,300), (10,600), and (10,100) ppmv, respectively.
Has Missing Values?
No
Introductory Paper
By Irene Rodríguez-Luján, J. Fonollosa, A. Vergara, M. Homer, R. Huerta. 2014
Published in Chemometrics and Intelligent Laboratory Systems 130
Variables Table
Variable Name | Role | Type | Description | Units | Missing Values |
---|---|---|---|---|---|
class | Target | Categorical | no | ||
Feature1 | Feature | Categorical | no | ||
Feature2 | Feature | Categorical | no | ||
Feature3 | Feature | Categorical | no | ||
Feature4 | Feature | Categorical | no | ||
Feature5 | Feature | Categorical | no | ||
Feature6 | Feature | Categorical | no | ||
Feature7 | Feature | Categorical | no | ||
Feature8 | Feature | Categorical | no | ||
Feature9 | Feature | Categorical | no |
0 to 10 of 129
Additional Variable Information
The responses of the said sensors are read in the form of the resistance across the active layer of each sensor; hence, each measurement produced a 16-channel time series, each represented by an aggregate of features reflecting the dynamic processes occurring at the sensor surface in reaction to the chemical substance being evaluated. In particular, two distinct types of features were considered in the creation of this dataset: (i) the so-called steady-state feature (DR), defined as the maximal resistance change with respect to the baseline and its DR normalized version (DR divided by the acquired value when the chemical vapor is present in the test chamber). And (ii), an aggregate of features reflecting the sensor dynamics of the increasing/decaying transient portion of the sensor response during the entire measurement. This aggregate of features is a transformation, borrowed from the field of econometrics and originally introduced to the chemo-sensing community by Muezzinoglu et al. (2009), that converts the transient portion of the sensor response into a real scalar by estimating the maximum/minimum value y[k] for the rising/decaying portion of the exponential moving average of the sensor response: y[k] = (1-Alfa) y[k-1]+Alfa(R[k]-R[k-1]) where R[k] is the sensor resistance measured at time k and Alfa is a scalar smoothing parameter between 0 and 1. In particular, three different values for Alfa=0.1, 0.01, 0.001 were set to obtain three different feature values from the rising portion of the sensor response and three additional features with the same Alfa values for the decaying portion of the sensor response, covering thus the entire sensor response dynamics. Thus, each feature vector contains the 8 features extracted from each particular sensor, resulting in a 128-dimensional feature vector (8 features x 16 sensors) containing all the features and organized as follows: DR_1, |DR|_1, EMAi0.001_1, EMAi0.01_1, EMAi0.1_1, EMAd0.001_1, EMAd0.01_1, EMAd0.1_1, DR_2, |DR|_2, EMAi0.001_2, EMAi0.01_2, EMAi0.1_2, EMAd0.001_2, EMAd0.01_2, EMAd0.1_2,..., DR_16, |DR|_16, EMAi0.001_16, EMAi0.01_16, EMAi0.1_16, EMAd0.001_16, EMAd0.01_16, EMAd0.1_16 where: DR_j and |DR|_j are the R and the normalized R features, respectively. EMAi0.001_j, EMAi0.01_j, and EMAi0.1_j, are the emaR of the rising transient portion of the sensor response for Alfa 0.001, 0.01, and 0.1, respectively. EMAd0.001_j, EMAd0.01_j, and EMAd0.1_j, are emaR of the decaying transient portion of the sensor response for Alfa 0.001, 0.01, and 0.1, respectively. The index j=1…16 represents the number of the sensor, forming thus the 128-dimensional feature vector. For processing purposes, the dataset is organized into ten batches, each containing the number of measurements per class and month indicated in the tables below. This reorganization of data was done to ensure having a sufficient and as uniformly distributed as possible number of experiments in each batch. Batch ID Month IDs Batch 1 Months 1 and 2 Batch 2 Months 3, 4, 8, 9 and 10 Batch 3 Months 11, 12, and 13 Batch 4 Months 14 and 15 Batch 5 Month 16 Batch 6 Months 17, 18, 19, and 20 Batch 7 Month 21 Batch 8 Months 22 and 23 Batch 9 Months 24 and 30 Batch 10 Month 36 Batch ID: Ethanol, Ethylene, Ammonia, Acetaldehyde, Acetone, Toluene Batch 1: 83, 30, 70, 98, 90, 74 Batch 2: 100, 109, 532, 334, 164, 5 Batch 3: 216, 240, 275, 490, 365, 0 Batch 4: 12, 30, 12, 43, 64, 0 Batch 5: 20, 46, 63, 40, 28, 0 Batch 6: 110, 29, 606, 574, 514, 467 Batch 7: 360, 744, 630, 662, 649, 568 Batch 8: 40, 33, 143, 30, 30, 18 Batch 9: 100, 75, 78, 55, 61, 101 Batch 10: 600, 600, 600, 600, 600, 600 The dataset is organized in files, each representing a different batch. Within the files, each line represents a measurement. The first character (1-6) codes the analyte, followed by the concentration level: 1: Ethanol; 2: Ethylene; 3: Ammonia; 4: Acetaldehyde; 5: Acetone; 6: Toluene The data format follows the same coding style as in libsvm format x:v, where x stands for the feature number and v for the actual value of the feature. For example, in 1;10.000000 1:15596.162100 2:1.868245 3:2.371604 4:2.803678 5:7.512213 … 128:-2.654529 The number 1 stands for the class number (in this case Ethanol), the gas concentration level was 10ppmv, and the remaining 128 columns list the actual feature values for each measurement recording organized as described above.
Dataset Files
File | Size |
---|---|
batch7.dat | 5.9 MB |
batch10.dat | 5.8 MB |
batch6.dat | 3.7 MB |
batch3.dat | 2.6 MB |
batch2.dat | 2 MB |
0 to 5 of 10
Reviews
There are no reviews for this dataset yet.
pip install ucimlrepo
from ucimlrepo import fetch_ucirepo # fetch dataset gas_sensor_array_drift_at_different_concentrations = fetch_ucirepo(id=270) # data (as pandas dataframes) X = gas_sensor_array_drift_at_different_concentrations.data.features y = gas_sensor_array_drift_at_different_concentrations.data.targets # metadata print(gas_sensor_array_drift_at_different_concentrations.metadata) # variable information print(gas_sensor_array_drift_at_different_concentrations.variables)
Vergara, A. (2012). Gas Sensor Array Drift at Different Concentrations [Dataset]. UCI Machine Learning Repository. https://doi.org/10.24432/C5MK6M.
Creators
Alexander Vergara
DOI
License
This dataset is licensed under a Creative Commons Attribution 4.0 International (CC BY 4.0) license.
This allows for the sharing and adaptation of the datasets for any purpose, provided that the appropriate credit is given.