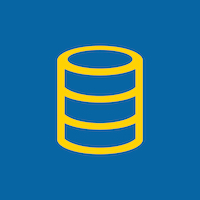
Pedestrians in Traffic
Donated on 7/3/2019
This data-set contains a number of pedestrian tracks recorded from a vehicle driving in a town in southern Germany. The data is particularly well-suited for multi-agent motion prediction tasks.
Dataset Characteristics
Multivariate, Sequential, Time-Series
Subject Area
Computer Science
Associated Tasks
Classification, Regression, Causal-Discovery
Feature Type
Real
# Instances
4760
# Features
14
Dataset Information
Additional Information
The raw data was acquired from a vehicle equipped with multiple sensors while driving, for approximately five hours, in an urban area in southern Germany. The sensor set included one mono-RGB camera, one stereo-RGB camera, an inertial measurement system with differential GPS and a lidar system. The preprocessed data available from this repository consists of 45 pedestrian tracks (in world coordinates) together with a semantic map of the static environment. For each track and at each time-step, not only the agent position is provided, but also body and head orientation attributes, as well as the position of all other agents and their type (e.g. car, cyclist, pedestrian etc.). Additional details about the preprocessing pipeline can be found in [1]. More information on the data format is provided in the next section.
Has Missing Values?
Yes
Variables Table
Variable Name | Role | Type | Description | Units | Missing Values |
---|---|---|---|---|---|
oid | Feature | Integer | no | ||
timestamp | Feature | Continuous | no | ||
x | Feature | Continuous | no | ||
y | Feature | Continuous | no | ||
body_roll | Feature | Binary | yes | ||
body_pitch | Feature | Binary | yes | ||
body_yaw | Feature | Continuous | yes | ||
head_roll | Feature | Binary | yes | ||
head_pitch | Feature | Continuous | yes | ||
head_yaw | Feature | Continuous | yes |
0 to 10 of 14
Additional Variable Information
: Pedestrian tracks are stored in the tracks.csv. Each row in such files contains 14 comma-separated attributes, with missing values denoted by ‘None’. The attributes are in order: • oid: unique agent id (int), • timestamp: time in seconds (float), • x: x component of position vector (float), • y: y component of position vector (float), • body_roll: roll body angle in degrees (float), • body_pitch: pitch body angle in degrees (float), • body_yaw: yaw body angle in degrees (float), • head_roll: roll head angle in degrees (float), • head_pitch: pitch head angle in degrees (float), • head_yaw: yaw head angle in degrees (float), • other_oid: list of ids of agents currently present in the scene ([list of int]), • other_class: list of other agents’ class labels ([list of int]), • other_x: list of other agents’ x coordinates ([list of float]), • other_y: list of other agents’ y coordinates ([list of float]). Labels used to identify agent types are available in agent_class_label_info.csv. The file semantic_map.png contains a map of the static environment, where semantic labels are color-encoded according to the mapping available in semantic_map_label_info.csv. Information needed to transform between image and world coordinates is stored in the file map2world_info.txt.
Dataset Files
File | Size |
---|---|
pedestrian_traffic_data/tracks.csv | 1.9 MB |
pedestrian_traffic_data/semantic_map.png | 307.2 KB |
pedestrian_traffic_data/semantic_map_label_info.csv | 148 Bytes |
pedestrian_traffic_data/map2world_info.txt | 140 Bytes |
pedestrian_traffic_data/agent_class_label_info.csv | 94 Bytes |
Reviews
There are no reviews for this dataset yet.
pip install ucimlrepo
from ucimlrepo import fetch_ucirepo # fetch dataset pedestrians_in_traffic = fetch_ucirepo(id=536) # data (as pandas dataframes) X = pedestrians_in_traffic.data.features y = pedestrians_in_traffic.data.targets # metadata print(pedestrians_in_traffic.metadata) # variable information print(pedestrians_in_traffic.variables)
Pedestrians in Traffic [Dataset]. (2019). UCI Machine Learning Repository. https://doi.org/10.24432/C5189C.
DOI
License
This dataset is licensed under a Creative Commons Attribution 4.0 International (CC BY 4.0) license.
This allows for the sharing and adaptation of the datasets for any purpose, provided that the appropriate credit is given.