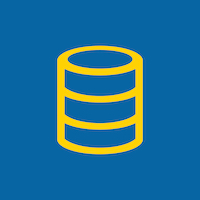
Rice (Cammeo and Osmancik)
Donated on 10/5/2019
A total of 3810 rice grain's images were taken for the two species, processed and feature inferences were made. 7 morphological features were obtained for each grain of rice.
Dataset Characteristics
Multivariate
Subject Area
Biology
Associated Tasks
Classification
Feature Type
Real
# Instances
3810
# Features
7
Dataset Information
Additional Information
Among the certified rice grown in TURKEY, the Osmancik species, which has a large planting area since 1997 and the Cammeo species grown since 2014 have been selected for the study. When looking at the general characteristics of Osmancik species, they have a wide, long, glassy and dull appearance. When looking at the general characteristics of the Cammeo species, they have wide and long, glassy and dull in appearance. A total of 3810 rice grain's images were taken for the two species, processed and feature inferences were made. 7 morphological features were obtained for each grain of rice.
Has Missing Values?
No
Introductory Paper
By Ilkay Cinar, M. Koklu. 2019
Published in International Journal of Intelligent Systems and Applications in Engineering
Variables Table
Variable Name | Role | Type | Description | Units | Missing Values |
---|---|---|---|---|---|
Area | Feature | Integer | Returns the number of pixels within the boundaries of the rice grain | px | no |
Perimeter | Feature | Continuous | Calculates the circumference by calculating the distance between pixels around the boundaries of the rice grain | px | no |
Major_Axis_Length | Feature | Continuous | The longest line that can be drawn on the rice grain, i.e. the main axis distance, gives | no | |
Minor_Axis_Length | Feature | Continuous | The shortest line that can be drawn on the rice grain, i.e. the small axis distance, gives | no | |
Eccentricity | Feature | Continuous | It measures how round the ellipse, which has the same moments as the rice grain, is | no | |
Convex_Area | Feature | Integer | Returns the pixel count of the smallest convex shell of the region formed by the rice grain | no | |
Extent | Feature | Continuous | Returns the ratio of the region formed by the rice grain to the bounding box | no | |
Class | Target | Binary | Cammeo and Osmancik | no |
0 to 8 of 8
Additional Variable Information
1.) Area: Returns the number of pixels within the boundaries of the rice grain. 2.) Perimeter: Calculates the circumference by calculating the distance between pixels around the boundaries of the rice grain. 3.) Major Axis Length: The longest line that can be drawn on the rice grain, i.e. the main axis distance, gives. 4.) Minor Axis Length: The shortest line that can be drawn on the rice grain, i.e. the small axis distance, gives. 5.) Eccentricity: It measures how round the ellipse, which has the same moments as the rice grain, is. 6.) Convex Area: Returns the pixel count of the smallest convex shell of the region formed by the rice grain. 7.) Extent: Returns the ratio of the regionformed by the rice grain to the bounding box pixels. 8.) Class: Cammeo and Osmancik rices
Class Labels
Cammeo and Osmancik
Dataset Files
File | Size |
---|---|
Rice_Cammeo_Osmancik.arff | 417.6 KB |
Citation_Request.txt | 2 KB |
Reviews
There are no reviews for this dataset yet.
pip install ucimlrepo
from ucimlrepo import fetch_ucirepo # fetch dataset rice_cammeo_and_osmancik = fetch_ucirepo(id=545) # data (as pandas dataframes) X = rice_cammeo_and_osmancik.data.features y = rice_cammeo_and_osmancik.data.targets # metadata print(rice_cammeo_and_osmancik.metadata) # variable information print(rice_cammeo_and_osmancik.variables)
Rice (Cammeo and Osmancik) [Dataset]. (2019). UCI Machine Learning Repository. https://doi.org/10.24432/C5MW4Z.
Keywords
DOI
License
This dataset is licensed under a Creative Commons Attribution 4.0 International (CC BY 4.0) license.
This allows for the sharing and adaptation of the datasets for any purpose, provided that the appropriate credit is given.