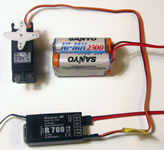
Servo
Donated on 4/30/1993
Data was from a simulation of a servo system
Dataset Characteristics
Multivariate
Subject Area
Computer Science
Associated Tasks
Regression
Feature Type
Categorical, Integer
# Instances
167
# Features
4
Dataset Information
Additional Information
Ross Quinlan: This data was given to me by Karl Ulrich at MIT in 1986. I didn't record his description at the time, but here's his subsequent (1992) recollection: "I seem to remember that the data was from a simulation of a servo system involving a servo amplifier, a motor, a lead screw/nut, and a sliding carriage of some sort. It may have been on of the translational axes of a robot on the 9th floor of the AI lab. In any case, the output value is almost certainly a rise time, or the time required for the system to respond to a step change in a position set point." (Quinlan, ML'93) "This is an interesting collection of data provided by Karl Ulrich. It covers an extremely non-linear phenomenon - predicting the rise time of a servomechanism in terms of two (continuous) gain settings and two (discrete) choices of mechanical linkages."
Has Missing Values?
No
Variables Table
Variable Name | Role | Type | Description | Units | Missing Values |
---|---|---|---|---|---|
motor | Feature | Categorical | no | ||
screw | Feature | Categorical | no | ||
pgain | Feature | Integer | no | ||
vgain | Feature | Integer | no | ||
class | Target | Continuous | no |
0 to 5 of 5
Additional Variable Information
1. motor: A,B,C,D,E 2. screw: A,B,C,D,E 3. pgain: 3,4,5,6 4. vgain: 1,2,3,4,5 5. class: 0.13 to 7.10
Dataset Files
File | Size |
---|---|
servo.data | 3.1 KB |
servo.names | 2.1 KB |
Index | 108 Bytes |
Reviews
There are no reviews for this dataset yet.
pip install ucimlrepo
from ucimlrepo import fetch_ucirepo # fetch dataset servo = fetch_ucirepo(id=87) # data (as pandas dataframes) X = servo.data.features y = servo.data.targets # metadata print(servo.metadata) # variable information print(servo.variables)
Ulrich, K. (1986). Servo [Dataset]. UCI Machine Learning Repository. https://doi.org/10.24432/C5Q30F.
Keywords
Creators
Karl Ulrich
DOI
License
This dataset is licensed under a Creative Commons Attribution 4.0 International (CC BY 4.0) license.
This allows for the sharing and adaptation of the datasets for any purpose, provided that the appropriate credit is given.