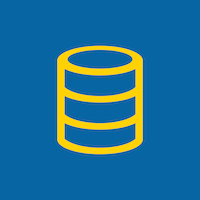
Simulated data for survival modelling
Donated on 12/3/2018
A variety of survival data, with carefully controlled event and censor rates, is available to allow people to develop and test new approaches to survival modelling.
Dataset Characteristics
Multivariate, Time-Series
Subject Area
Other
Associated Tasks
Regression
Feature Type
Integer, Real
# Instances
120000
# Features
25
Dataset Information
Additional Information
We generated two batches of data, where each batch consists of 20 datasets. For the low dimensional batch, we used 5 predictive parameters, of which 2 were dummy parameters (i.e. had no impact) and three were predictive. For the medium dimension batch, we used 25 predictors, of which 2 were dummy and 23 predictive. In each batch, we varied the event rate from 10% to 70% and the censor rate from 0% to 70% in 20% steps, and used a set population size of 3000. This therefore led to two batches, each of 20 datasets of 3000 subjects.
Has Missing Values?
Yes
Variable Information
For the low dimensional batch: x.0 & Binary: with equal probabilities x.1 & Gaussian: μ = 50, σ = 15 x.2 & Uniform: [1,2,3,4] x.3 & Binary: 0.6 chance of 0 x.4 & Uniform: [1,2,3] For the medium dimension batch: x.0 & Binary: with equal probabilities x.1 & Gaussian: μ = 50, σ = 15 x.2 & Uniform: [1,2,3,4] x.3 & Binary: 0.6 chance of 0 x.4 & Uniform: [1,2,3] x.5 & Binary: 0.95 chance of 0 x.6 & Binary: 0.9 chance of 0 x.7 & Binary: 0.85 chance of 0 x.8 & Binary: 0.8 chance of 0 x.9 & Binary: 0.75 chance of 0 x.10 & Binary: 0.7 chance of 0 x.11 & Binary: 0.65 chance of 0 x.12 & Binary: 0.6 chance of 0 x.13 & Binary: 0.55 chance of 0 x.14 & Binary: 0.5 chance of 0 x.15 & Binary: 0.5 chance of 0 x.16 & Binary: 0.45 chance of 0 x.17 & Binary: 0.4 chance of 0 x.18 & Binary: 0.35 chance of 0 x.19 & Binary: 0.3 chance of 0 x.20 & Binary: 0.25 chance of 0 x.21 & Binary: 0.2 chance of 0 x.22 & Binary: 0.15 chance of 0 x.23 & Binary: 0.1 chance of 0 x.24 & Binary: 0.05 chance of 0
Dataset Files
File | Size |
---|---|
MLtoSurvival-Data/25 Covariates/50E_10C_3000N_25Cov.csv | 342.7 KB |
MLtoSurvival-Data/25 Covariates/70E_70C_3000N_25Cov.csv | 270.7 KB |
MLtoSurvival-Data/25 Covariates/50E_70C_3000N_25Cov.csv | 268.9 KB |
MLtoSurvival-Data/25 Covariates/70E_50C_3000N_25Cov.csv | 268.3 KB |
MLtoSurvival-Data/25 Covariates/30E_70C_3000N_25Cov.csv | 265.8 KB |
0 to 5 of 42
Reviews
There are no reviews for this dataset yet.
pip install ucimlrepo
from ucimlrepo import fetch_ucirepo # fetch dataset simulated_data_for_survival_modelling = fetch_ucirepo(id=581) # data (as pandas dataframes) X = simulated_data_for_survival_modelling.data.features y = simulated_data_for_survival_modelling.data.targets # metadata print(simulated_data_for_survival_modelling.metadata) # variable information print(simulated_data_for_survival_modelling.variables)
Simulated data for survival modelling [Dataset]. (2018). UCI Machine Learning Repository. https://doi.org/10.24432/C57G99.
DOI
License
This dataset is licensed under a Creative Commons Attribution 4.0 International (CC BY 4.0) license.
This allows for the sharing and adaptation of the datasets for any purpose, provided that the appropriate credit is given.